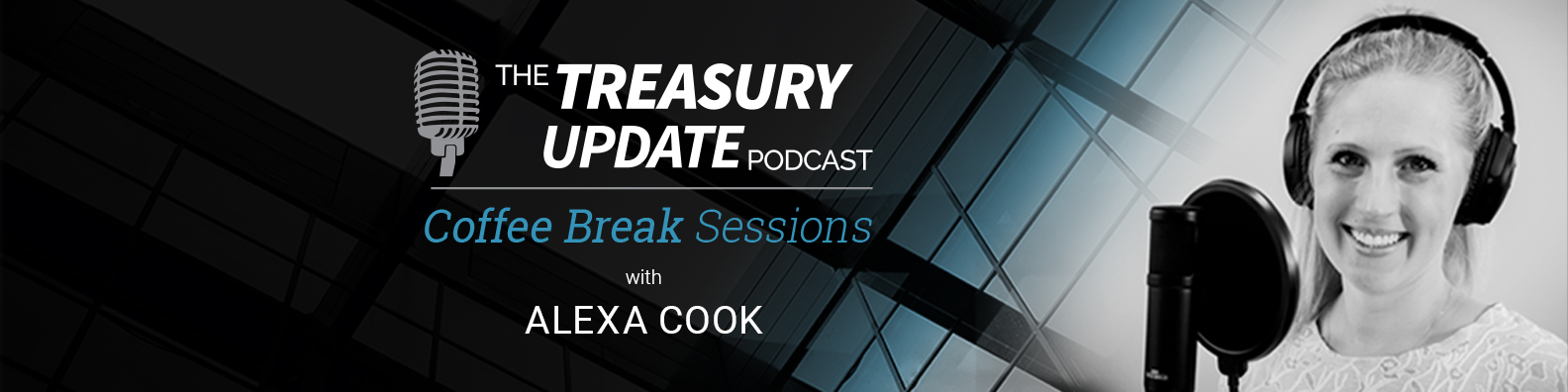
Session 12
What is a Data Lake?
What is a data lake? Coffee Break Session Host Alexa Cook joins a Zoom meeting with Strategic Treasurer’s Managing Partner Craig Jeffery to discuss data lakes. They provide an overview of data lakes and describe its different uses. Tune in and learn a little bit about data lakes.
Host:
Alexa Cook, Strategic Treasurer
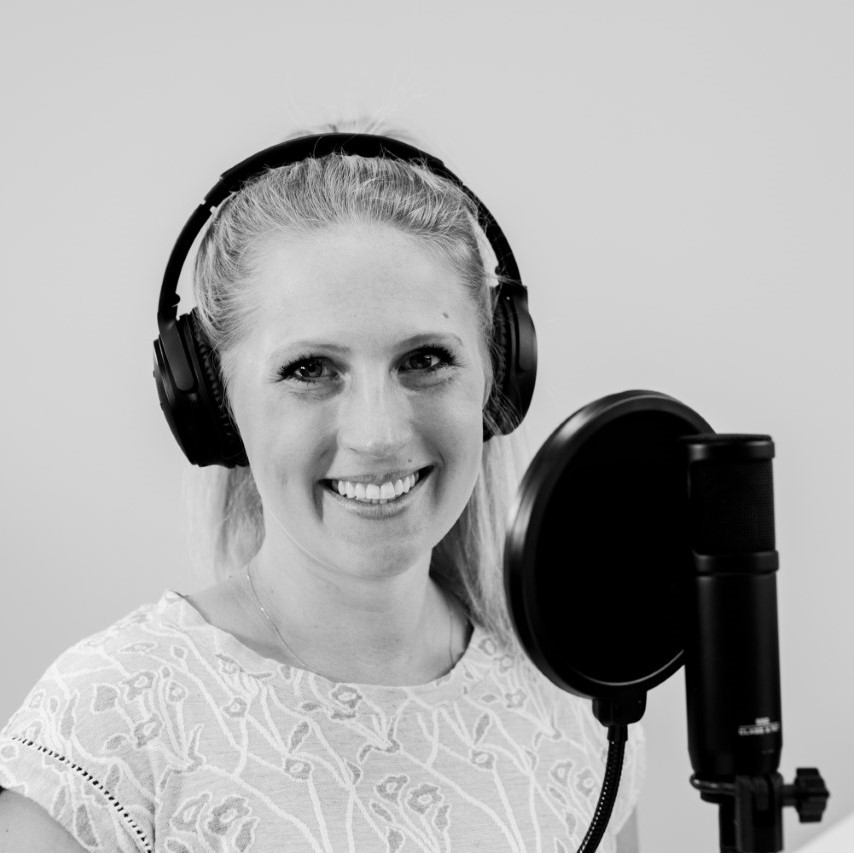
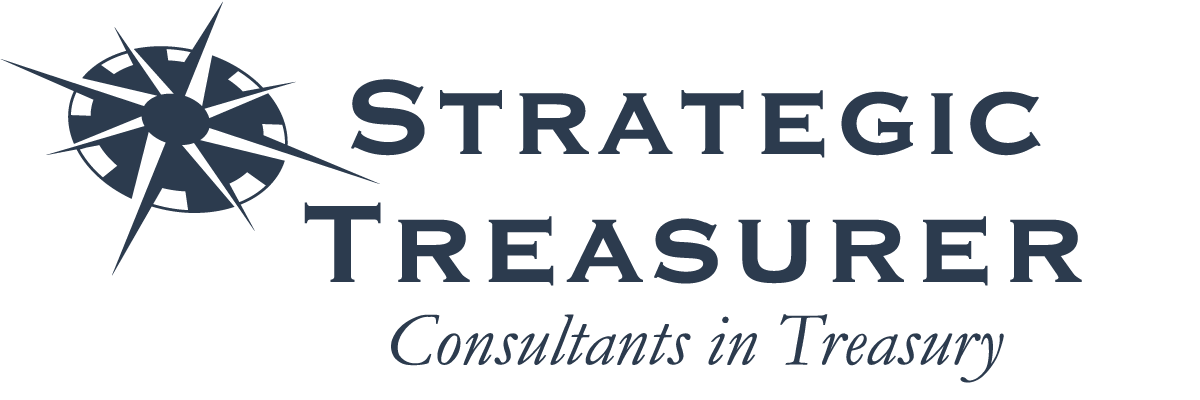
Speaker:
Craig Jeffery, Strategic Treasurer
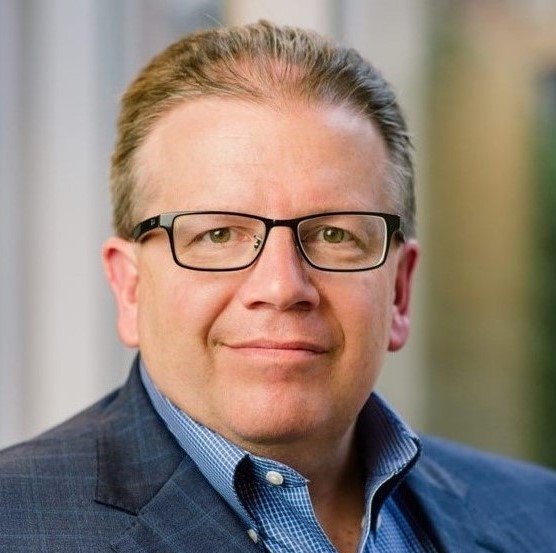
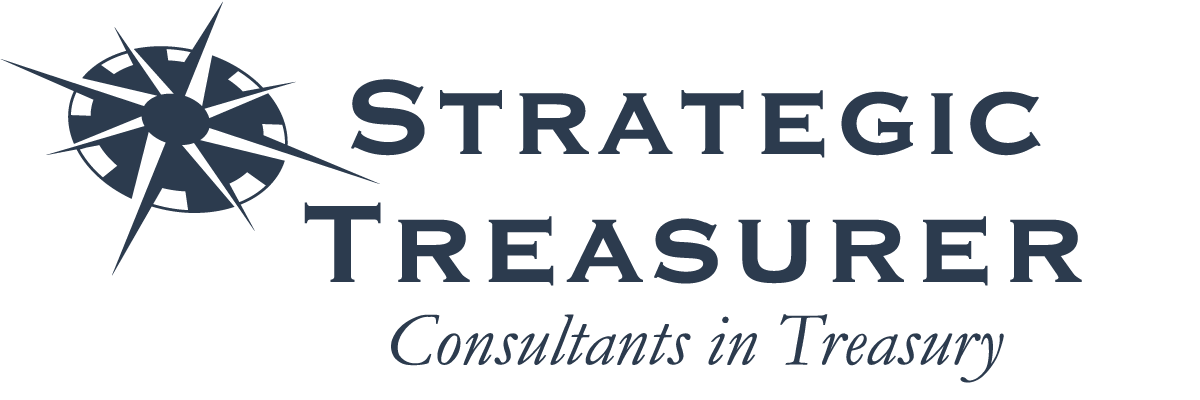
Episode Transcription - CBS Episode 12: What is a Data Lake?
Alexa Cook:
Hey guys! Welcome to the Treasury Update Podcast, Coffee Break Session, the show where we cover foundational treasury topics and questions in about the same amount of time it takes you to drink your coffee. Today, we’re going to be talking about data lakes and just data in general and big data. And Craig, I think you’re the perfect fit for this because you are very knowledgeable on this topic. So why do we care about data?
Craig Jeffery:
So I mean, people care about data for a couple reasons, but the biggest one for treasury is not just that it relates to transactions and activity. Though, that’s really important. That’s always been important. So we care about data now, I think, because it allows us to generate better insights, perform more deep analysis, see correlations that we couldn’t see before. So we care about data because of what we can do with it.
Alexa Cook:
Okay. That makes sense. What is a data Lake then?
Craig Jeffery:
So yeah. So if you talk about how we store data and how we access it. Data lake is a cool, modern term. Data lake is a, let’s call it a repository, where you can dump or pour data in that is both structured, semi-structured, and unstructured. And I think that’s pretty much a universal understanding. That’s certainly how we think about it. Structured would be it’s in a database format. It’s an Excel file. It’s table-driven. Semi-structured might be XML, extensible markup language related. It might be some kind of delimited format, like CSVs, things like that. And unstructured data might be memos, reports, webpages, video, audio with tags, et cetera. And a data lake is a place where you can pour all of this information in, and then you can analyze. You can use your BI tools, your other database tools, to do some analysis, achieve additional insights, pull things together from disparate formats, and start asking that next level of question, which leads you to more questions. So a data lake is that big repository for all types of data.
Alexa Cook:
Okay. That was my next question. Is it all types of data or can you just dump anything in? And it sounds like through the three different types that from Excel or the structured version, even to the unstructured version, which was more email or PDF or less machine readable things. So it sounds like a lot of it can be just dumped in.
Craig Jeffery:
Yeah. Yeah. So poured in, since we’re trying to use the liquidity theme here, like pouring it in versus dumping it in, you wouldn’t necessarily throw everything in there because of the mass quantities of data that you have. I think we know that data is growing about 40% a year, which means it doubling every two years. And so from everything from, we have more data points coming into our financial systems, all the way to the internet of things, capturing all kinds of readouts, whether it’s supply chain, et cetera. There are massive quantities of data that continues to grow. A data lake doesn’t mean whatever you have anywhere, throw it in there. It has to be moved into there or poured into there in some logical manner.
Craig Jeffery:
But it doesn’t have to be as structured or end goal in mind, like a data warehouse might be, where a data warehouse is, I know what my questions are. I determine what information I need for that. I determine the source of that information. And then I build a model to pull that data out of my accounting system, my point of sale system. I move it and structure it, stick it into these, this warehouse. I create subtotals and structure. So now I can run all my reports and queries against this fully structured data warehouse. We don’t have the complete end goal in mind. We just know we need to be very flexible with what we’re trying to do. We have to have an adaptable outcome in mind. In other words, we’re going to have questions that come up that we don’t know that we have those questions at the beginning. As we learn, we need to answer more questions.
Alexa Cook:
So it’s not all encompassing, just the amount of data there’s too much of it, but it could be all encompassing if you wanted it to be.
Craig Jeffery:
I mean, it’s going to grow. There’s no question that you’re going to put more and more data in there. It’s easier to query. You can load more of it in memory. Petabytes seem massive today, but we’ll probably be carrying that around on our phones in 15 years. There’s massive amounts of computing power and massive amounts of storage that continue to scale just like our data grows and scales.
Alexa Cook:
You kind of touched on it already, but I did want to maybe differentiate a data lake from a data warehouse or a database and a data mart. And I think you’ve pretty much already answered how a data warehouse differs and that’s you really what the end result is. So if it’s a cookie, then everything that you’re putting in is going to be the flour, sugar, eggs, all of that, whereas a data lake, you might not know if you want like a cookie or a candy bar. So what you’re pouring into it is going to be different, right, than what the data warehouse would be.
Craig Jeffery:
Yeah. That’s exactly right. Yeah. So I mean, data cubes and data warehouses are you have a lot of stuff you need to do regularly and repeatedly. Think about finance or FP&A, there’s people have a lot of questions that they’re going to ask every quarter, every month. And so a data cube or a data warehouse would be the types of things people leverage, and they’re great. They’re great tools for those types of purposes when you know the outcome.
Craig Jeffery:
But you asked what’s the difference between a database. So database is a organization of tables, typically a relational database. For example, they’ll have a bunch of tables with keys that link different tables together on key fields. If it’s employee records that might have employee number or employee ID, and that links address information to pay roll to other elements, and they’re stuck at different tables, so that it’s nice tight tables, but you can do your processes and you can run other reports without filling out this massive matrix that’s 15,000 columns and so many rows for each person because of all the different options. So it could be benefits they have for health, for dental, et cetera. So a database is an organized structure designed around a single type of data or process that’s managed, related, and efficient and usually uses relational concepts to be more efficient.
Alexa Cook:
How is this tying back to big data? Are data lakes helping with that? Because when I think of big data, I’m thinking of just everything, kind of how I alluded to data lakes being all encompassing. So how does that help or how do those two tie together?
Craig Jeffery:
When I think of big data, I think of all of the amounts of data that exist and are being created. So this can be internal financial data, operational data, transactional data. It’s everything that’s being populated from news media reports, information on the markets, interest rate curves, et cetera. All that information is just there’s massive amounts of data. The concept of big data recognizes that we need to know some of that data, whether it’s very structured data, like we talked about in a data lake, or whether it’s all the way unstructured, it’s a video clip of a news report on a bank, or what’s the effect of a possible pandemic on production facilities in an Asian country.
Craig Jeffery:
So all of that stuff can flow together because if you said, “Hey, I want to know about my counterparty who’s building stuff,” I may would need to know where are they located? What’s the outlook? What other news elements are impacting our business with them? We’re used to doing a Bing search or Googling a particular topic and looking up and say, “Here’s pictures of it. Here’s news press release. Here’s something on Wikipedia. Here’s their homepage,” and you can gather and consume that data in different formats. And so big data says, “There’s a lot of data out there, internal and external data, private data, public data, data we can buy.”
Alexa Cook:
Okay. How does that relate or matter for treasury? Would treasury departments use data lakes or, and how? And who is doing that? Because I feel like this is all relatively new as far it comes to the treasury world.
Craig Jeffery:
It is relatively new and it’s not just a fad. This is something that will continue and grow. And some treasury groups are using it, and some IT groups are certainly provisioning data lakes and leveraging those. So for example, if you need to stand up a data lake, you could do a lot of work to set it up on your own, or let’s say you’re an Office 365 company, or even if you’re not, you can provision an Azure data Lake from Microsoft in minutes, stand it up, put volumes, and then start populating that. I mean, obviously there’s some more design to it, but same thing with Amazon Web Services. IBM and Google have services, that in the cloud, allow you to provision a data lake. I do think treasury cares about it. Data lakes, the use of BI tools are all part of Treasury’s goal and responsibility to look at risk, to forecast properly, to understand the implications and correlations of what’s in the market. And given that, they need to be able to do better analysis.
Craig Jeffery:
Well, let me start with one example. And if I think of another one I’ll change that to two, but let’s say one example is counterparty risk management. I want to understand what my exposure is to main counterparties, the biggest ones, so anything over $10 million. Well, what might my exposure be to banks? I could have a credit facility. I might have a bank balances in 25 accounts. I may have investments that have, as the holder, my CUSIP set might be related to a particular bank. I could have car transactions that relate to a bank, et cetera, et cetera, that could be a customer buying some services. So I just gave five examples.
Craig Jeffery:
All of that information that I listed isn’t going to sit in one system in a company. So the customer data is not going to sit in a treasury system. The credit information, the counterparty might be sitting in a treasury management system. Your investments might be sitting in a custody platform. You might have one or two admin systems that are keeping track of the other elements, maybe a point of sale system. So now you have data in multiple places. So a BI tool or a dashboard that sits over a single system does a good job of looking at what’s in that system. It doesn’t do as good of a job, and the wallet’s changing, it doesn’t do as good of a job of accessing lots of other sets of data to pull that together.
Craig Jeffery:
So the counterparty risk example is, “Hey, stuff is in a lot of places. I need to pull it over, organize it, and calibrate it.” So after you pull it over, you might say, “I also want some ratings. I want NRSRO ratings to see what those ratings are from Moody’s or S&P.” Maybe you want some market related data. So this might be credit default swap rates on your banks, just to see what the market is thinking about the three year, the 18 month CDS on those particular items, so you can calibrate that particular exposure.
Craig Jeffery:
So treasury would care about that because they need to have a reasonable view of counterparty risks, not just banks, but maybe banks, key customers, or suppliers because there’s some risk management that they need to do. So you have to have the data gathered, normalized and organized in a way to then apply your risk factors and look at that. And given how many systems that are out there, this is a big issue.
Alexa Cook:
So I feel like we’re a bit over on time, as far as the Coffee Break lengths typically go. So I’ll try to recap it. Data lakes are really repositories where all kinds of data are poured in. I think you went through the three different types where it was structured, semi-structured, and unstructured. Data lakes are important for treasury, because as you just said, through those examples, you can pull all kinds of information from all different types of sources to look at risk or forecasting or just deeper analysis. And especially with data, I think you said it was doubling every two years. I think that it’s really important to kind of have treasuries starting to think about moving towards or pouring into a data lake.
Craig Jeffery:
Yeah. I think that’s really accurate. And just made me think of the one other phrase I would say is that why do you care about this? And why do you look at this? And this recognizes that concept of you don’t know the end question, when you start. it’s recognizing that you will get smarter as you look at data and will ask more questions and you won’t know those questions at the start. Therefore, you won’t have the data, unless you think of the process differently. I need to make sure I have all the important data. I connect it. I reference it. I associate it, so that I can ask these tables that go beyond what a warehouse or a data cube can get me today.
Alexa Cook:
Okay. Is there anything else you want to add on data lakes?
Craig Jeffery:
No, but it is a cool term and it is exciting to see some companies starting to use it in treasury. Some of it’s playing around some of it’s some key examples that they’re working on it. And I expect that to grow quite rapidly. This is a smart area for at least one person in each Treasury area to work with their IT that’s working on a data lake and get moving on.
Alexa Cook:
Yeah, we’ll have to revisit this topic maybe a year’s time to see how it’s changed since it sounds like this might be a rapidly changing area as far as IT and Treasury goes, but thanks for joining us today, Craig. And for all of our listeners, make sure to tune in every first and third Thursday of the month for a new podcast recording. And if you have any comments, questions, or just want to get in touch with us, we can be reached podcast@strategictreasurer.com. Thanks.
OUTRO:
Podcast is provided for informational purposes only and statements made by Strategic Treasurer, LLC on this podcast are not intended as legal, business, consulting, or tax advice. For more information visit and bookmark strategictreasurer.com.
Related Resources
Want access to more great content? Subscribe to Strategic Treasurer on YouTube!
A part of the Treasury Update Podcast, Coffee Break Sessions are 6-12 minute bite-size episodes covering foundational topics and core treasury issues in about the same amount of time it takes you to drink your coffee. The show episodes are released every first and third Thursday of the month with Special Host and Treasury Consultant Alexa Cook of Strategic Treasurer.