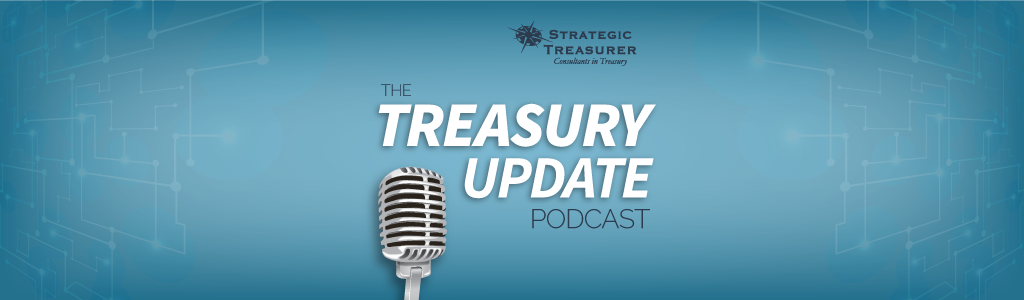
Episode 187
Open Banking APIs Enhance Analysis Through Machine Learning-Powered Search
Host:
Craig Jeffery, Strategic Treasurer
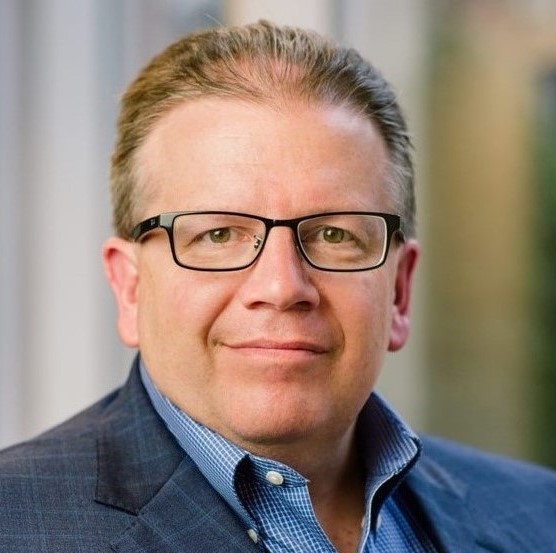
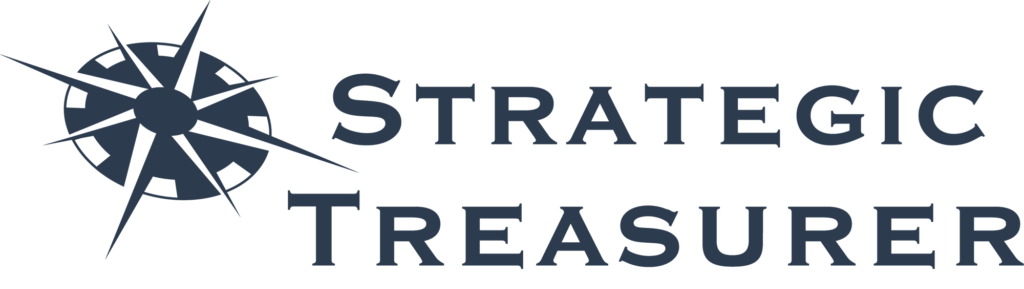
Speaker:
Joseph Drambarean, Trovata
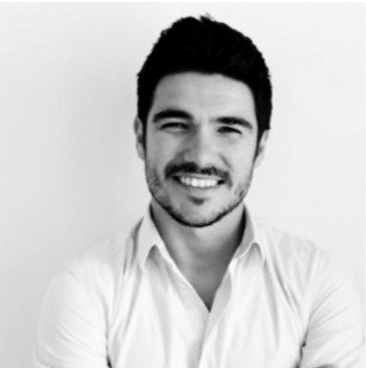
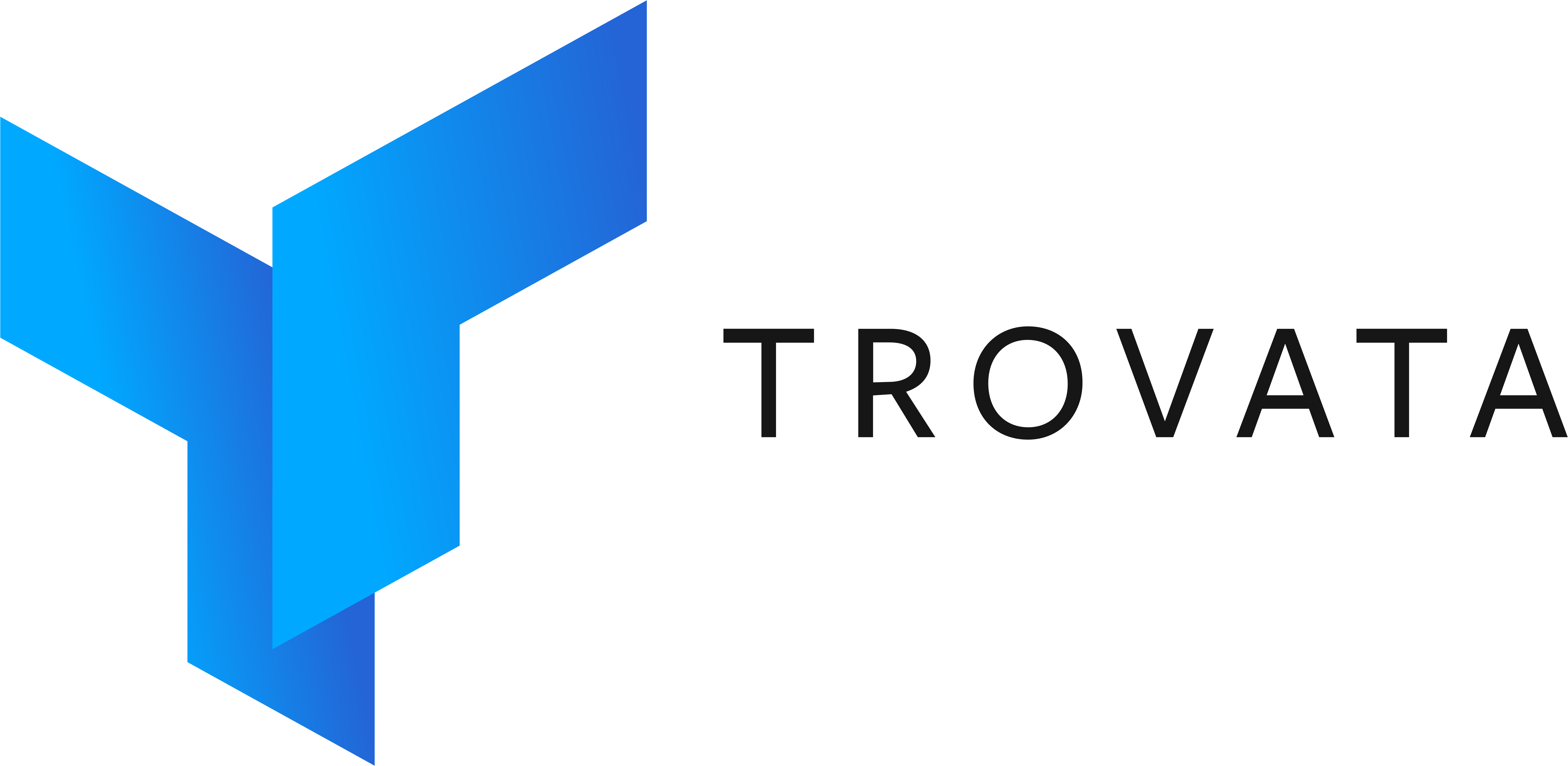
Subscribe to the Treasury Update Podcast on your favorite app!
Episode Transcription - Episode #187: Open Banking APIs Enhance Analysis Through Machine Learning-Powered Search
INTRO 0:04
Welcome to The Treasury Update Podcast, presented by Strategic Treasurer, your source for interesting treasury news, analysis, and insights in your car, at the gym, or wherever you decide to tune in. On this episode of the podcast, host Craig Jeffery sits down with Joseph Drambarean, Chief Technology Officer at Trovata to discuss how open banking API’s enhanced financial analysis through machine learning powered search. They share valuable insights on how your organization can break free from the grid by reducing manual workflows through automated data aggregation. So, you can focus on strategic analysis that drives value for your business. Listen in to the discussion to find out more.
Craig Jeffery 0:54
Joseph, welcome to The Treasury Update Podcast again.
Joseph Drambarean 0:57
Hey, Craig, it’s great to see you again and always happy to be on the podcast. I’m excited about today’s topic.
Craig Jeffery 1:03
Before we get into the topic of open banking API’s and how, you know, machine learning powered search is enhanced by that, maybe you can just give us a quick review of your background and role at Trovata. I’ll drop a link in the show notes that links people to the previous podcast, but if you just give us a quick overview, we’ll start with that.
Joseph Drambarean 1:23
Yeah, of course. I’m the Chief Technology Officer here at Trovata and I’ve been involved at Trovata, really from the beginning of the company. We’ve been trying to innovate in the space of cash management, really, for the last five years now. And we’ve taken an approach of using some of the best practices in cloud computing, machine learning and just a non-traditional approach to data when it comes to cash management. It’s led us down a road of building software and infrastructure for some of the biggest companies in the world, whether we’re talking to companies like Square or Etsy or Fastly, or Sonos, or RingCentral and using technology to drive automation and drive brand new use cases within the realm of forecasting and cash management. So, I’ve been in charge of the technology organization since day one. I really manage them, the product, as well as the infrastructure and technology underpinning that product.
Craig Jeffery 2:21
Since we’re gonna be talking about machine learning powered search. I wanted to start with search and how has search progressed and how that changes us. And I’ll give a little bit of a story to start out with I don’t I don’t know how old you are. Joseph, I’m not asking you that. But I do remember going to the library where they have these file cards for searching one was by like title and it was by author, and they were paper copies you have to look at them and they give you the code and you hunt through the stack to find the stuff. That was terrible. It was terrible then but that was all that existed. So how has search progressed? Maybe in the in the more modern area of computers? What is that doing to change how we think and how we act?
Joseph Drambarean 3:05
I think that’s called the Dewey Decimal System. If I’m not mistaken, maybe I’m wrong about that. But yeah, it’s it. Things have come a long way. Ironically, that’s actually a very efficient system. The problem is, is that it’s based on qubits. Qubits are the processing capacity that gives you access to information in that system. And really, what’s happened, obviously, when we talk about search, and we think about search, one of the first things that comes to mind is Google search, right? It’s our archagent behavior of going to google.com. And anything that comes on our mind whether it’s looking for a specific thing, or trying to find a file or whatever it might be within our life, you can use that one bar, you know to put in your thoughts in non-structured or structured form, and it’ll find what you’re looking for either the answer to a question, or a link to a website or a piece of content like an image or a video, whatever it might be. Well, that is actually the result of an evolution that’s taking place in computing. Computers have always been really great at finding things. It’s actually one of the most basic things that a computer can do within the structure of a file system or within a database. The problem has always been how can you infer the right types of context to power a search that is actually useful? But that’s where the evolution has happened. You know, we had systems like, you know, AltaVista, or Ask Jeeves or Google, all of these or Yahoo search. We had that at the beginning of the internet, right and that was the makings of the internet 1.0 if you will, you know, the internet that caused the bubble. Brought everything to its knees. And what was missing in that time was this added metadata that makes search so powerful. That makes us so reliant on it today. And the metadata comes from the addition of context that provides clarity into what it is that you’re looking for, so that the computer can be more efficient in searching the right databases across different indexes to get your answer is the equivalent of having a librarian that is aware of that same Dewey Decimal system, but knows that when you’re asking for a work of fiction, that she or he is going to look at a specific index as opposed as opposed to putting it all together and going one by one and looking at every single one in that array. And that’s really what’s changed over the last few decades is we’ve not only found more efficient ways to index different parts of the internet, but we’ve added context, we’ve added metadata that comes from your interaction with the internet, right? So, we all are online now we have online presence. We have calendars that are connected to online profiles, we have itineraries that are connected online profiles. We have networks. We have folks that that are connected to us that are safe things that we are sharing things with, and that normal relationship across all these different services and data types and interactions that we have create this meta data sphere, if you will, that when combined and organized in a specific way can create extremely powerful search, which is what happens when we go to Google search, unbeknownst to us, you know, from the time that we’re young. When we go to Google, we just type in our thoughts. what Google is doing under the hood is using machine learning to try to whittle down that thought into a likely intent, the thing that you’re trying to find and then based on that puts you into the right work stream to find the results of that query in the appropriate database that it’s maintaining on its side. So, it could be all the internet crawling that it’s been doing, trying to find websites that are applicable. It could be an answer that they’ve been collecting through Wikipedia. They’ve been assembling that on their side. It could be a YouTube video, that is a perfect match for a thing that you’re trying to learn. It could be an email that is within your Gmail, but you’re just happened to be searching within the Google search bar. And because it’s identifying that intent, it knows, “Oh, you know what, let me look inside of their email before I surface other results”, because it knows that the context is close. So, that has actually changed our behavior, I’d say, a pretty fundamental way. Our reliance on search as a culture has driven us to not only be able to discover knowledge more efficiently, but also has created behaviors whereby which we can branch off into different types of knowledge search, that is more wisdom than if we were trying to collect that information, you know, from the root level. And what I mean by that is there are all kinds of thoughts that emerge in your mind when you can find things easily. Let me give you an example. Let’s say that I’m trying to plan a trip to Los Angeles. And it starts with how do I get to Los Angeles, right? If I find that the best way to get to Los Angeles is to take the 5 and then from there, you know, take the Coastal Toll Road that might branch a new thought of saying, Well what are what are some things to do near the coast, which, if I have access that information quickly, I might find you could actually go to this beach and use it as a stopping point before you continue on your journey to LA. And that might trigger a thought of what are the best restaurants in that coastal town that I just found. And that meandering that ability to let one thought lead to another all powered by search that gives me an answer in a low latency way. It creates a completely different type of behavior when it comes to planning when it comes to comes to problem solving. And it also when it comes to just learning in general, and I guess to bring this topic to a useful financial analogy, I guess this is the type of behavior change that we have found very interesting, that extra bottom if you could have a very similar mindset when it comes to traversing financial information, finding things first and foremost. But then once you have found them, having a deep understanding of the context of why it matters, the thing that you have found, and how it plays out over history and how it plays out over the future. Would it change your behavior? Would it make you operate in a different way? And could it create the type of mental elasticity that makes you a more strategic thinker within the role of Treasury or was it the role of planning or even at the CFOs office? And this thought of search is kind of the underpinning of all of it. Kind of how we went through this example of starting at the most simple thought of I need to get to LA or I need to open an office in Dublin. How would I go about that? You know, looking at the different streams of costs, the different streams of sales, all the information that you need to make a well-judged decision on whether or not you can open an office in Dublin. The mechanics by which you arrive to that kind of information we have found can be closely tied to the same mechanics of search that we’ve all become so used to when using products like Google or Bing or any other search tool that is available to us. Siri is another example that uses voice activated search. So that’s been really interesting it I think that that this this topic is so critical to the transformation of finance, generally, because one of the most painstaking aspects of financial analysis is knowing that you’re looking at the right information. And of course, that means that you have to get the right information in the first place, process it, make it ready for analysis. And in the process of doing that. You strip out a lot of the opportunity to have those widths of moments, if you will, the ones where you realize I could stop in Laguna Beach and have a nice afternoon at this restaurant, and it would only really shave off two hours from my journey. And that insight could lead to something else. And I feel like that’s where the future of financial data transformation lies. It’s in this new behavior and this behavior that sits on the shoulders of more strategic thinking because you have the leverage of finding information and finding insights in a way that was never before possible. And of course, the obvious future, even to that vision is you don’t even have to do any of that thinking. And that’s of course what Google has done to us, right? The more that we have searched the more we’ve input into Google system, they have enough context to just start to predict to just start to realize, “Oh, you’re going on a trip to LA here’s a full itinerary. Here. Let me tell you all the best places to stop”. Don’t even search I know. I know. What your behavior, behavioral characteristics are the things that you would like to see, give it your profile, the interactions you’ve had in the past things that you’ve searched things that you bought, and it’s that kind of seeking that although creepy and sometimes you know, it’s something that you know, we could have a completely different discussion about that context, drives the necessary assumptions to drive leverage at the point of decision making. And it’s a concept that we take very seriously when we think about the journey that a person finance-oriented planning or even a CFO is taking because once we know enough of what you’re looking for, the types of analysis that you’re doing the types of search that you’re doing, the things that you’re worried about. It gives us the footing to start to predict and say, “This is where you could be, this is what we see and what you should do about it”. And it is, you know, obviously a wave change in behavior.
Craig Jeffery 13:53
The search, obviously, search continues to learn and get smarter. This context aspect, sometimes you can see it’s not sure it’s like “Did you mean… Did you mean this?” And I and I remember I’ve tried to remember how many years ago it was it was more than five years ago, the phone I had, I was at a hotel going to a client. And what was interesting was my phone buzzed and said, “You should leave now to get to your appointment”. I never set it up that way. It had the address in knew when I was supposed to go there. It was traveling by car, and it looked at traffic and said you need to leave now because there’s some delays. And you we need to get you there 15 minutes early. I never set up any of those parameters. And so, it made it smarter, and I sort of remember first being shocked a little bit ticked off that it just my computer read my calendar and knew where I was. I was like that’s really useful. But you know that idea of context. Joseph, as you talked about this smarter search, you know, and this idea of metadata. I want to I want to talk about that for just a moment. You know that part of that I think is when you ask a question, you get smarter when you get the answer and the less time you spent hunting for stuff. The more you can I think use the term winsome. The more can be a happy experience for figuring these things out. Is this the idea of now I can say I can look at my forecast. And now because there’s metadata is other data associated with it that I can find? Who’s paying me what type of payment is what type of account it goes into. I can learn more I can explore more I can self-discover more. Is that what you mean by like, it just gets it helps with context, which helps me make it smarter.
Joseph Drambarean 15:47
Context is such an interesting topic because it has active and passive parts to it. The active parts are of course, when you’re in the moment and you’re trying to figure something out. The joy parts of context are you don’t have to do that much work because the system is so in tune with your thoughts, that it just surfaces the right thing for you. And the example that you gave is a classic homerun example of context driven interactions where calendar, time, traffic equals an incredible moments, right? Creepy, but an incredible moment where it has saved your meeting. It has literally gotten you there on time because it made a recommendation insight, right? There are very similar things that can exist within the realm of finance, right? There are very predictable patterns when it comes to cash flow. Things that happen on a cadence basis, whether it’s payroll, whether it’s disbursements that are related to loans or things of that nature, those pieces of context that if unearthed from the DNA of your cash flow, if you will, they become a platform on which we can start to use tis base assumptions. And as you’re searching, let’s say that you’re looking for something specifically, let’s say that you’re typing in
payroll, right? Because we are aware of the cadence intervals of payroll and the specific elements of your cash flow, that are your cash flow that line up with payroll, we can start to do intelligent things like group payroll together as a concept and payroll might be comprised of ADP. It might be comprised of a few other vendors that are used Gusto, that all roll up to payroll, you think about payroll in one phrase, we know that payroll actually means eight different line items that all line up to meet payroll to you. And because of that inference, we could turn the analysis of something as, it seems simple payroll, but it is actually complex when looking at it from a financial statement perspective, because it arrived at all that data, you have to use the right search terms, you have to be looking for the right cadence and you have to have the original financial statements in the first place from the banks to be able to get that data. So, if we are able to wrap that all up into a quick, “Oh, I just type in P-A-Y-R”. Instantly it suggests, “Are you looking for payroll?”, that becomes a moment where not only are you feeling the delight, actively, in that search moment, but you can start to build off of that. Now that you know, “Oh, I can find things by just typing in P-A-Y-R” and it knew I was looking for payroll. I wonder what else it can find. And that leads to discovery that leads to a change of your behavior instead of focusing on trying to collate that data just right, getting it ready for the insight that would have been payroll, you’re just off to the races and you’re starting to do analysis. You’re starting to wonder; I wonder what our payroll looked like over the last 90 days. And it could be as simple as tuning the graph to see the last 90 days and those types of interactions when you get used to them just like we got used to search over the last, you know multiple decades of experience with search it changes the way that you interact with your computer. In fact, search is not something that I would describe in my day to day as a burden of experience. It’s something that is so incredibly, I don’t think about it at all. Let’s put it that way. Okay, we even talked to our phone, what we search, right? You hold down your button and you use Google assistant or Siri and you say, “Hey, how many points did Steph Curry score last night?” And that’s actually a complicated search. There are multiple elements that search the first thing is who is Steph Curry? In the context of points, what are you talking about? Is he an athlete? Is he not? All that so works out all of those mechanics by itself. And the simple answer of well, he scored 28 points last night. That’s that is accurate too by the way, I think. That is a complex answer that’s been arrived through that through context. But you didn’t know any of those things. You just wanted to know how many points did he score? The fact that you’re used to that, that you’re used to that kind of answer being given to you changes your behavior. And in the context of this example, let’s say that I was downstairs, and I was talking to a group of friends, and we wanted to settle a debate because nobody actually remembers how many points he scored. And you want it to just say hey, I can figure that out. Let me ask Siri and Siri tells you that. Now you are continuing on with your conversation. You can have your argument, right? And it turns into an interaction in the same vein. Let’s say that you’re sitting in a pressure moment, whether you’re in a boardroom setting, or you’re in a setting where you’re meeting with a supervisor and there are questions around specifics around the cash flow. That would take maybe a week to figure out if you had to organize that data, find it put it together and present it in a meaningful way. Would your behavior change if you knew in the back of your mind, I can find all the answers that this supervisor is asking for within seconds by just typing in phrases and things that are coming to mind in the moment. Would you be more fluid in your interactions? Would you be more strategic in your advisory because you knew you could lean on a system that has context and search built into its foundation? I believe that the answer is yes. You would change your behavior. And now I know that the answer is yes from experience with customers that have told us so. And that’s really what we’re getting at here is that if you build a platform that is focused on these types of objectives what is the result? Where does it take us as a financial industry? Do we make better decisions? Do we make faster decisions? Ultimately, it remains to be seen I’d say, but the early insight that I have is that it is changing behavior and it comes down to the foundation of these mechanics and I think that’s why it’s so interesting.
Craig Jeffery 22:32
A couple of things came to mind as you were talking to those one was that I can’t remember it was like a Saturday Live skit about someone saying how many did Satchel gets last night. Satchel Page has been dead for forty years. It was like it was it was hilarious about people not having the right context on the computer was correcting that. But even that’s a good example. And you know this, this idea of what would it be like if you could get the data, you needed served up in the way you wanted? I think that’s I think it’s powerful because historically, it was always hard to get at the data. We were missing things from making a lot of assumptions. While I think there still can be some challenges with do you have all of the data across all of your organization, are you not making assumptions, it’s gonna be faster and easier to tell and to check some of these items out. As opposed to saying I need to build everything from step one to step 50. And if there’s any change in the question, I got to go back and redo, maybe all of those steps maybe most of those steps, now I don’t have to do that because the structure outset. But you know, part of that, Joseph is the components now you’ve used a bunch of terms like meta, metadata. What’s necessary to make this type of environment happen? When I say this type of environment, I think it’s part of I think we might need to explain what is the “this” what’s needed, what isn’t needed. And there’s obviously some elements of I would assume search that is necessary for this context. You have to have data stored in a place you can get it right; it just can’t be separate unsearchable items. And then, you know, you mentioned the term data and metadata, you know, this enriched information that allows you to, whether you call it tagging it or just associating it, this is what provides the context. So, what’s necessary for this type of search and this type of context? To make it work. Did you have to have the components are the best search engine if it can’t get the data can’t do it?
Joseph Drambarean 24:42
That’s absolutely right. And I love the example that you brought up because it’s so easy to extrapolate from that example to a real-world example in financial transactions. So, in yours, you had a meeting that you had to get to. And if we wanted to create an analogy here, the fact that the meeting was taking place at you know, 1234 Acme Street in New York, New York. That was the description of the transaction, if you will, it’s the thing that everybody will always have. But that isn’t telling us enough to know whether or not you’re going to make it there on time. Right? That actually doesn’t tell you any of that. The fact that you are going to take a car to get there is a really important piece of information. You’re not taking a plane, a helicopter, you’re not using a jetpack, you’re using a traditional mode of transportation, and you’re going to take it in a way where you’re susceptible to road conditions, right? So, an assumption was made there. In the world of financial transactions, I can make an analogy to that of the rail that’s being used to process that transaction, could be a wire, it can be an ACH, it can be a variety of different means. And the context of that rail means something it could provide detail that is important to the overall transaction that was settled. Now there are other pieces of information time, the fact that you knew that you wanted to be there 15 minutes in advance, right? You knew that that was an important element to the story of being at that meeting on time. In the calendar invite, the meeting takes place at 10:30am. So just judging by the description of that meeting, you would know that your preference is to be there early so that maybe you could clear your mind. Maybe you need to use the bathroom before jumping into the meeting. Maybe you need to freshen up whatever it is that will make you successful in that meeting, that metadata that contacts is an important factor in making the declaration that leave now so that I can take into consideration the buffer of traffic and the time that you want in advance putting that together to get to me to be successful when getting to that meeting. That information if I were to make a loose analogy, the additional contacts, remittance data, for example, or data coming from a source system, like an ERP system, giving context of this being for example, a disbursement for a specific vendor or whatever it might be. That context tells us a lot about what the urgency of that payment might have been looking like, why a certain rail was used, why a fee structure was applied to that particular payment versus a different payment. All that information can be used to discern a picture of almost like the DNA of that transaction, why it took place where it took place. How often will it take place again? Is this an anomaly? Is it normal? Is this a transaction that should be flagged as fraud? Those are pieces of information that are only surfaced through broader pieces of information coming as a constellation around that core description. Right? Every bank statement will give you the description of this was you know a wire of number 071162. And what are you supposed to do with that? You know that? It’s great. It was a wire. Thank you. You know, I noticed that I made this wire transfer on August 3. You need more information to know that that wire was actually a disbursement for an investment. That is for a specific company that is in the Philippines and that company that you’re making an investment in the Philippines for it actually has a tie in to a forecast item that is principal to the growth forecast for this next two quarters. That tight correlation of information is really marrying data that’s coming from the big with human intelligence that’s being put into a system, right? And that’s the best of every world, right, when you can take different systems create context that stitches them together, and surface them through powerful search. And that’s exactly what Google does. That’s what happened with your example. That kind of experience, it takes planning from a technology perspective to execute on that kind of experience. You have to have the right data first and foremost, right? We mentioned it if we didn’t have any one of those elements from your calendar example. We wouldn’t have gotten an effective prediction of when we should leave. We needed all of it. And that’s why Google and Facebook and now called Meta they care so much about every aspect of the contextual model of your day-to-day life. It’s because the more they have, the more accurate their prediction and their search will be when you’re looking for something or what you’re trying to do your next thing in the same way when we’re trying to make predictions about the outcome of a financial blind item, or anything related to finance. The more information that we have to establish past, present, and future, the better and more accurate we can be. And the data that we get, a lot of it comes from make transactions, but a ton of it also comes from human generated metadata. So, information like I know that we want to grow by 2% this quarter or I am putting in this invoice as a payment and I know that it’s going to pay on the 23rd. These are pieces of information that while yet not fully manifested, not actual transactional record. They are meaningful data points that tell us about where things will go. And therefore, they are related to ultimately a search outcome. If we tie them all together, and we find the appropriate associations to them, then we can create these types of experiences. And I think that’s why what I refer to when I when I use the word metadata, it’s these tangential but extremely relevant elements of information that are nesting ultimately to a transactional record.
Craig Jeffery 29:43
So, they’re associated with it, but they may not be in the original file that came with it the first part of the data. So, an XML record the extensible markup language has an element, that element by itself has no context, what’s the header, or what’s it may say that’s the transaction amount. So, it tells you that it’s the transaction amount that also comes within a file that tells you that the date it tells you other information, there might be other elements that are associated with that that tell you like you said, payroll or something related to payroll, and they can build an infer from these other associates and links just like your brain, all the connections that get built. So, you can think it’s perhaps the same way with the data. The metadata is how I learn and associate other things with other things that can help the system respond in a way that’s contextualized or smart.
Joseph Drambarean 30:40
Right. That’s a great analogy, because ultimately, when we make decisions when we have thoughts, it’s based on a relational graph of information that one thought might be about a specific thing, but it’s influenced by memories, emotions, a variety of other factors that when collected together, lead you to that thought, in the same way, all information and it’s naked form is information without context, but as you attach notes to it, and you form a graph of information that ultimately still has a pivot point, it has a parent that is influencing that graph. The elements of that graph, the nodes that comprise it all create a more crystallized picture of why it is what it is, where’s it going? And you can use that for inference.
Craig Jeffery 31:31
One of the elements about how do we get accurate information? We have to clean data. How do you have clean video to clean up underlining systems, and that’s what you people usually say. And that’s almost never the case. When you look at all the various systems that are it’s not standard. It’s different. Bank of America is listed as B of A, Bank of America, Bank of America BAML, Merrill Lynch. And so, you can say I’ve got to fix all the underlying data and every other line source. I don’t know that it’s not possible. I’ve just never seen it work, but you can have associative tables or whatever that repair that and so, hey, all the bad stuff underneath is wrong, but you have a fixed table that you know, as it learns, it fixes it and you said when I say B of A or Bank of America, it knows that it’s Merrill Lynch, Bank of America B of A, however it’s stored originally. And this metadata or associative tables helps repair it so you get what you need.
Joseph Drambarean 32:32
And there’s no skipping that hard work. That is something that every major social network company has to deal with, right? If they’re pulling data from different subsystems, advertising systems, from mailing campaigns, from apps that you’ve given access to them to, it’s not going to be in a standard format, right? Almost assuredly, it will not be. So, there has to be a transformation step to that data ingestion. That creates the appropriate naming conventions for some of these things that takes guesses at some of them so that it can be useful at index time and that search time. A very similar thing is something that we do at Trovato that you can’t cut around, right? There will be there will never be a magical company that just says, “Hey, give us your data. We’ll just wink wink and it’ll work”. You actually do have to transform it; you have to make it useful for the purposes of indexing and all of that. So, the exact process that you were talking about is exactly what happens. You make assumptions about the fields, and how they should be ported over, and you create a unified format, one that takes all of the common denominators from all the different formats, and then uses the rest of the data in an unstructured way to still give you the context that you need to be able to do the search. It’s painstaking and it’s trial by error for the most part, but once you get at that common model, is when the fun starts, is when you have that speed and efficiency of search. And it’s something that, of course, we’ve been investing in for a very long time now. And it’s really pivotal to the whole agenda. That is why it is called data transformation.
Craig Jeffery 34:26
So, Joseph, with the beginning of the title of this podcast is called Open Banking API’s Enhance Analysis. How do open API’s help with this process of super smart contextual search that helps us in finance and Treasury?
Joseph Drambarean 34:46
There’s two ways that it will be helpful and that it currently is helpful. I’ll start with the currently helpful. APIs are not burdened by the same technological limits that were in place 20 years ago, 30 years ago when data standards were put into place, taking advantage of limitations to computers in those times, which means that an API driven data model might have no limits when it comes to characters that are delivered through a single payload of data that is delivered. And as a result of that, the data can be named differently. There can be more data of more context relating to that transaction. And the record itself can be cleaner. And when I mean cleaner, I actually do mean from a human readable perspective. The information can be a long form, it can provide addresses, it can provide PII information that could be useful downstream for reconciliation. It can provide a variety of different attributes that are coming from the source, for example, if it was a batch payment, maybe the originating line item that initiated that batch would make its way into the payment itself. These types of data types, let’s say that it arrived at a payload of over 30,000 characters in one record, that wouldn’t be a problem, right? Because using standard compression, we can send JSON files up to you know, 15 megabytes in size through a standard RESTful API call, right? So, we don’t have to worry about how big the data will be when we receive it, meaning that we can provide more data in these formats. Now, of course, the problem is that as the realization has started to matriculate through the industry, everyone has arrived at their own format, right? And while there are dramatically more pieces of metadata that are included in open APIs, now a new problem has been created, which is standardization, which is why that process of transforming that data and making it useful, so important up front. Now over time, just like SWIFT and BAI standards and others, created a unified format that banks agreed upon when providing information reporting, the same will happen in open banking APIs. In fact, it already has started to happen in Europe and in Asia. It’s already a standardized format when it comes to open banking API’s. In the US, it’ll probably take a little bit more time, but it will get there and it’s only a matter of time. That will be good for everyone. Because having a unified format that’s metadata rich that provides information and context that’s necessary for machine learning activities and search activities is how data transformation and the future of finance happens. We are at a stage now where we have to use technology to circumvent the drawbacks of different formats and computers are really good at this. So, the good news is that we don’t have to worry too much. It’s just a work that we have to do right now, to supplement the eventuality of the format being unified. But it doesn’t mean that we can’t have the benefits of search today.
Craig Jeffery 38:29
That’s a good point. I did have a question on the unified standards are consistent. If everything becomes unified, or standardized, there’s some benefits of this whole process of transforming the data is faster because it’s already done, but if don’t we want to have more enriched data coming in over time so they can do more things. Things are, you know, considered now, you know, and in different ways. So, is there always going to be this tension of we want to be consistent and flexibility or there’s always going to be this replacement of better standards with richer data?
Joseph Drambarean 38:67
That’s a great question. I take a stab at answering it by saying we create our own problems over time. Just like we get used to an idea like search and search you know, took a lot of standardization on the internet to arrive to where we are today to get the benefits of HTML formatting and the way that websites are built so that they can be crawled effectively. And the technology evolution that took place to be able to do that over time as Internet standards changed. There was a ton that went into creating this the scenario that we have today. And of course, what did we do? We responded by creating a bunch of other metadata. New content content, whether it’s blogs or texts or tweets, or documents or videos or images, whatever you might want to create. We are doing it right and adding even more content to the internet in an unstructured way. In the same way I think that that tension will exist within data transformation from a finance perspective. Right now, we’re talking about a reality where if you’re able to search for things and create ad hoc reports and be able to do analysis, it’s such a seamless way that you’re not even thinking twice about it. Well, that’s going to create a new problem, right? You’re going to be creating so many of those things have so many different formats that have so many different types of contexts. It will lead yet another evolution, right, that ties that human generated metadata to the real financial records and creates sense out of all of it, and relevancy out of all of it. That kind of tumultuous rolling over of innovation is something that I’m excited about personally, because that’s how we get to a better version of what we do, a better version of ourselves even. Its rolling around on top of new plateaus of opportunity, just like the internet is nowhere near the same way it was 20 years ago. The same will be true when it comes to financial planning and analysis.
Craig Jeffery 41:20
I think we’ve come up with a better name for this podcast would be From Winsome Search to Tumultuous Data Management. Joseph anything else on the open API front before we head to our closing question.
Joseph Drambarean 41:37
One of the things that we kind of touched on a little bit but is really mission critical to the future of the Internet is the notion of privacy. And what it means to have access to all this data. One of the things that I’ve been keeping a close eye on with regards to open banking standards and the development of banking technology is how the end user has control over the data that they get access to, and how they can cut off that access at any point in the future. It’s something that we talk a ton about when referring to our own privacy right when it comes to our financial information or our health data or our search data or you know, even our social network data, how that data is still managed by us and controlled by us is it a really important aspect of modern computing. I think the same is true with regards to financial data, and as we get better and better at accessing more types of metadata and creating associations of that metadata back to originating records. Having fine grain control over who has access to that is going to be a really important pillar of this innovation, because ultimately, it represents the DNA of a company.
Craig Jeffery 42:57
That’s a really good point. That’s the principle of least privilege, right? Who needs to be able to access it? Who can control it? As we wrap up today’s podcast, today’s discussion, any final thoughts for us?
Joseph Drambarean 42:72
I think that ultimately, when we talk about search or innovation in the context of data transformation within finance, it’s really useful to keep your eye on the prize of why we’re doing it. Right. It’s so easy to get caught up in the different buzzwords that are out there, whether it’s machine learning or AI or analysis and all the benefits that you get out of it. But the point of it all, the reason why we’re doing, it is so that we can be better at our jobs, so that we can be better stewards of the finances that we’ve been privileged to oversee or to provide strategic direction in a world of chaos, right? As we live through COVID. We continue to live through it. There’s a tremendous amount of uncertainty. Technology should be bringing mechanics and experiences that help dilute the effects of that uncertainty. And that’s what I tried to keep as a northstar, as we think about products, and Trovata is ultimately it’s not just about throwing technology against the wall and seeing what sticks. It’s about creating experiences that actually transformed the lives of real people. That make their lives better, that make their lives more enjoyable in their day-to-day work. And ultimately, that’s how I feel about search. I think I’d be the first to stand on my table and say that my life would be way worse if I didn’t have search in it. If I didn’t have the ability to find things on a moment’s notice on any topic. I feel very strongly that that should be the case with the world of finance, and it’s a shame that it isn’t.
OUTRO 45:02
You’ve reached the end of another episode of The Treasury Update podcast. Be sure to follow Strategic Treasurer on LinkedIn, just search for Strategic Treasurer. This podcast is provided for informational purposes only, and statements made by Strategic Treasurer LLC on this podcast, are not intended as legal, business, consulting, or tax advice. For more information, visit and bookmark strategictreasurer.com.
Related Resources
Visibility, data connectivity and forecasting are crucial to staying afloat in today’s climate, and leveraging new technology is becoming more and more crucial to achieving those goals. From RPA to AI and ML, the alphabet soup is confusing but increasingly important to treasury’s daily life. Learn the fundamentals of these technologies and how they can empower your treasury team to unlock efficiency and access accurate information.
How Real-Time Payments Revolutionize Cash Management
Host Craig Jeffery joins Joseph Drambarean, Chief Technology Officer at Trovata, to discuss how real-time payments revolutionize cash management. Their conversation covers the concept of immediate payments, the areas where payment innovation is happening, the importance of driving trust, and the payment revolution that is underway for the next generation.