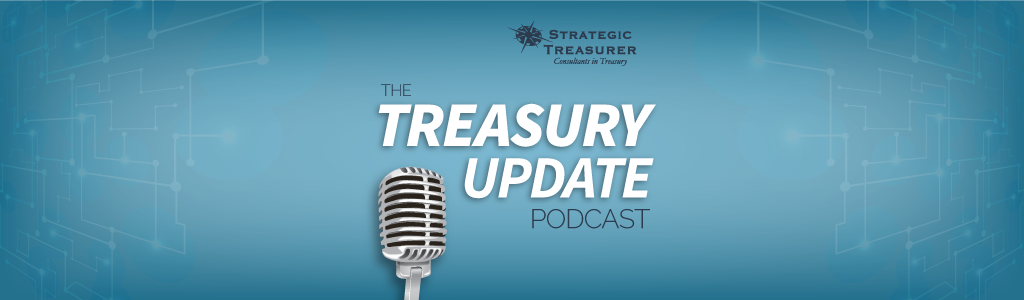
Episode 242
2023 Outlook Series:
Will AI Change Your Hiring?
Innovations like ChatGPT are changing the way we gather information. Will these advancements negate certain treasury roles in the future? Will it affect whom you hire and the size of your team? Craig Jeffery of Strategic Treasurer and Todd Yoder of Fluor discuss a couple of items to keep in mind as we move forward.
Host:
Craig Jeffery, Strategic Treasurer
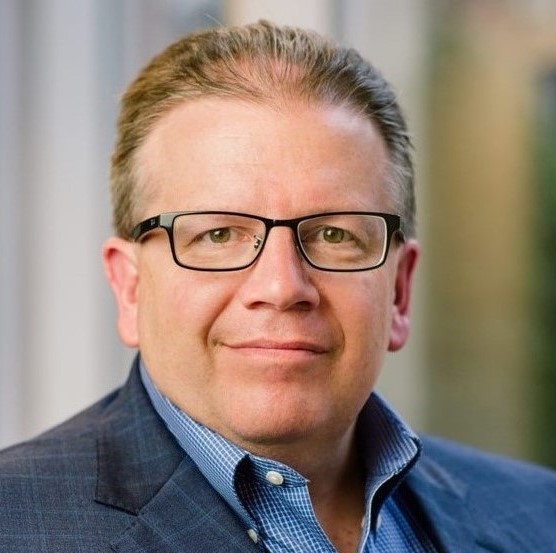
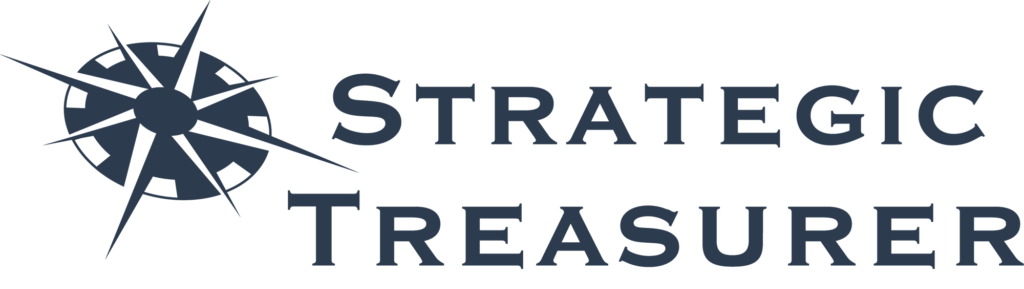
Speaker:
Todd Yoder, Fluor Corporation
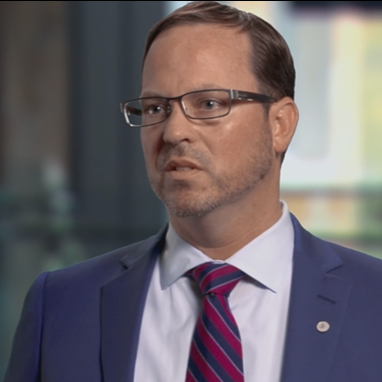
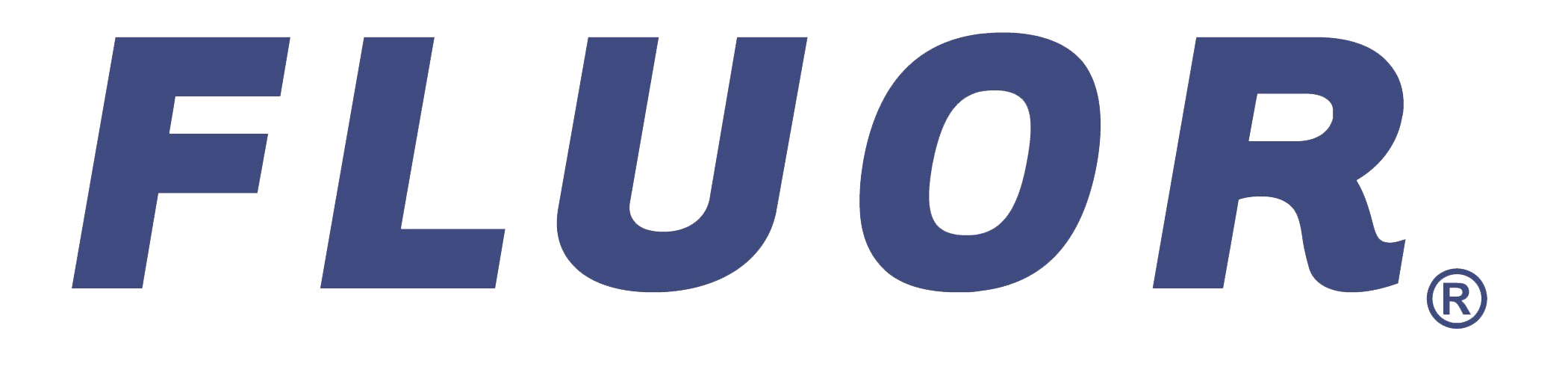
Episode Transcription - Episode #242 - 2023 Outlook Series: Will AI Change Your Hiring?
Announcer 00:04
Welcome to the Treasury Update Podcast presented by Strategic Treasurer, your source for interesting treasury, news, analysis, and insights in your car, at the gym, or wherever you decide to tune in.
Craig Jeffery 00:19
I can’t think of a better place to be in beautiful sunny Orlando, considering I spoke to my wife yesterday, and it’s cold in Dallas, Texas. It’s great to be here and always fun to chat Treasury with you. Welcome to the Treasury Update Podcast. This is Craig Jeffery, I am your host today back in the breakout room at an event we’re at in Orlando, Florida with Todd Yoder. Todd, would you give a quick introduction of yourself? I know you’ve been on the Treasury Update Podcast several times before. Yes, we had a good event the last couple of days. And today’s topic is part of the outlook series. And it’s the future of Treasury. I want to explore with you a few of these ideas that we’ve kicked around at different points. Not getting into super futuristic mode but but touch on some of these topics. And maybe before we talk about things like AI and machine learning, chat GPT, what happens with staff and Treasury? How will that change? What do we need to look at? I wanted to just talk for a few moments about the context of, of Treasury and hear your thoughts. I also had a few thoughts as well. I think we’re all seeing a recognition of increased volatility. Inflation has, has risen, central banks are trying to tamp down inflation. So rates are rising, we see, you know, ongoing volatility with FX but also with commodities. And it seems to me and from what we’re seeing is Treasury’s role has been elevated since the last financial crisis of 2008 and 2009 has stayed high and has had additional respect of late with forecasting, expectations placed on that more challenges, what are some of the things that you would see in terms of the expectations on Treasury or some of the new requirements in Treasury?
Todd Yoder 02:08
Treasury, you know, we saw in the global financial crisis crisis, Treasury got elevated, and more and more corporations, treasurers getting a seat at the table to be a big part of those conversations, what’s happening with the business, as I say, businesses are just like humans, every one of them are different with the volatility, and like you said, In the currency markets, interest rates, and commodities, and even logistics, right, and shipping in containers and the prices there, they’re having substantial impacts on the financial result. And so that is where the board of directors and CEO, you know, where they want to ring up the treasurer? And, in get some answers to that, what are we doing about it? And what are the thoughts? And can you put together a presentation for the board to get to walk them through it, you know, where we’re at and why we’re here? And, you know, and I think a lot of it is for the last, let’s say, 20, 30 years, you know, at least let since the global financial crisis, right, we’ve been into this low to no interest rate environment. And I think it’s brought along with it a lot of complacency, which is humanly natural. You know, when things are steady, and you don’t see a lot of volatility in the markets, you get comfortable. That’s one thing that you and I know you can never get comfortable, never get complacent. It’s constant learning, constant growing.
Craig Jeffery 03:49
The recruiter never should maybe that’s what you’re right. It’s like if it’s like, rates are low, money is cheap. That doesn’t. That’s not one of your first few items to look at. And but the future changes that some good points there the volatility, improving recognition and expectations. But I wanted to shift and talk a little bit about AI and machine learning. You know, I guess I guess I’ll give some background as like, what technologies are going to change Treasury the most. When I think about machine learning, the ability to detect patterns, there’s a lot of things happening in Treasury already. Anything that can detect patterns can be good for quality control, tracking and monitoring fraud, you know, anomalous behavior, it can help with forecasting and in certain ways, it’s this guide, and there’s lots of room to grow there. Those are all underway, in a sense, but, you know, we think about things like Chat GPT is pretty big of late. You type stuff in it gives them some type of answers. What are you thinking about AI and machine learning in the future? How is that going to impact treasurers? You may have some stories about Chat GPT. I’ve got one as well, but.
Todd Yoder 05:01
It’s a hot topic right now. And I think it’s a very important critical topic, I think it’s important to kind of give an overview, just for our treasures, and especially some of the younger treasures, I would first say there’s nothing like a human, the way we’re set up, the way we function, the way our organs work, you know, we are a being that is far superior, in holistic view, than any machines ever.
Craig Jeffery 05:34
So take that chat GPT.
Todd Yoder 05:36
So take that, from the start, I think I did my first Python, so machine learning demonstration, at Euro Finance in Copenhagen back in 2019. I first spoke on it and 2017, when it was about conversation about RPA, and about machine learning, and about how AI was going to impact. And back in 2017, I had no idea the progression, the acceleration. And as you and I talked about a lot, right, we boil it down to, you have the storage capacity to store data, it’s gotten cheaper and cheaper to store an insane amount of data. And then you have data creation. So the tools to capture data, in the amount of data that everyone started to realize five years or longer, you know, this data is valuable, the value of the data that we create as a business every year has an extreme amount of value. So we build these mega projects, but this data is very valuable. So and then it’s the compute power. So thanks to our friends at at Nvidia. And we know Chris treasurer of of Nvidia, great guy, you had CPU, then they invented GPU, and you have TPU. So the compute power is just, it’s expanding, accelerating like crazy. And then dev DevOps. So these developers, we are next generation of leaders that are younger than than you and I closer to Jonathan’s age, they’re brilliant, and they are developing stuff that is just amazing. So from an AI perspective, you know, how I feel about AI. I mean, I don’t like the term I like machine learning, Artificial Narrow Intelligence. But when you say ai 95% of the population thinks of ASI or AGI, you know, artificial super intelligence or AI, like it’s behaving and can act like a human, which is not, we’re not there. But I’ve given in, and I’ve accepted just we’ll call it AI. So, but really, you know, it all started 2012 was a really big period where you had the CNN, and CNN, not the TV station, but Convolutional Neural Networks, which did amazing work with image recognition. And for us and treasury, optical character recognition, this is where, hey, Treasury, you get all of these invoices bombarded into your AP department. And they may come in a paper form, they may come in, in email, they may come in a PDF, they may come in a Word document, you never know how those are going to come in. And there’s a lot of systems for collecting that. But OCR you know, optical character recognition really gave us the ability to build technologies that would go in and decipher all that and put it into our ERP language for our specific business. Then along came RNN. So the recurrent neural network that was more in the, you know, natural language processing. So the NLP, the problem with that, I mean, it solved a lot of things. But it was slow. When you really wanted to go out and scrape a lot of data. RNNs were just they just weren’t really fast and really good at that. So sequence is a big issue, that RNNs had trouble with sequence. And so then we fast forward to 2017. What really changed everything was a paper written by and I won’t say their names because I’m mispronouncing them, but it was about attention in the importance of attention in machine learning. And so that’s what created the transformer and then the transformers is what is all over the news. And so that took RNN but made it super fast. So then you could train on a bunch of data and you could drink quickly. So chat GPT is the one that’s all over the news. But there’s also BERTs and there’s T5, these are generative models. There’s another one that I follow. That’s not really big into the news, but Stable Diffusion, a young man from India, who’s a genius. He’s behind stable diffusion. And I love the guy because because those other models aren’t open source, Stable Diffusion, stable AI, is open source, he’s released it. And one of his big goals is education. So they can scrape two terabytes worth of data. And they can, they can get that down to they can run, run the machines, they can get it down to two gigabytes, they can put it on an iPhone, a laptop, tablet of some sort. And his goal, they can distribute it right to developing countries where they don’t have the privilege of education, as a lot of us do. And so his estimates were 13, an hour a day, for 13 months. Read and Write, can teach reading and writing. To a young person using that which education is a key to, to me is one of the huge keys. And you know, I’m big on the United Nations and 17 sustainability goals and education for children, you know, is really important to me. But then you have like chat GPT, which is basically 45 terabytes of data. It’s trained on 45 terabytes of data, which is essentially all of Web 2. I can’t even think in terabyte, 45 terabytes of data. And it’s scraping all of that data, right. And then it’s generative AI. So these transformers are generative AI. And that’s what we’ve all been playing with for the past few months is chat GPT. And Stable Diffusion is open source, but it’s really more text to image is their focus right now. But it’s definitely going to impact treasury.
Craig Jeffery 12:16
Yeah, so So on the generative, well, especially generative image, I mean, it’s, it’s fun to say, make a football player, right, an ostrich running across the surface of a moon or something that’s like, and it creates an image that’s like, well, that’s better than I could draw. It’s kind of odd. But it’s, you know, it’s the ability to put those things together. When you look at something like Chat GPT, and we think about treasure, what is what is going to help us and we already talked about some of the things that are already underway in Treasury. And so the the power of the tools to manage some of that activity for things like natural language search, the ability to scale, those are very, very helpful tools, far more advanced, or they’re, they’re at least a step above, when I think of some of the things that Treasury has to do is much more analysis, risk management. Looking at the future, it’s not just capturing data about the past. And so when I think of what jobs are going to be displaced, we already see that in things like audit jobs, where you’re piling through data, looking at patterns, detecting issues. By matching stuff, the consulting firms, audit firms are putting massive amounts of effort there, because it is faster than you know, the Army’s audit, you still have people guiding the activities, monitoring it, but they’re setting these tools is like, instead of having 50 people dig with a shovel, you put these tools out there, and it’s a giant earthmover. And it’s far faster than the mass of people, it still needs to be guided, directed, managed and summarized. But it’s displacing a lot of people in some of those fields. Treasure is not staffed heavily, even though we’ve seen, you know, over the last, you know, at least eight years, Treasury groups, on average, have been adding in 12 to 18, or 20% of those groups have added people every year. That’s the its general growth is still been very thinly staffed. So how is it going to impact this is kind of the to your question about what will go on. If you asked me 10 years ago, and 15 years ago, I always had this idea that it would be great if we could, you know, do cash positioning, forecasting, funds, movements, a lot of the core cash management activities in the treasury domain, if you get set that up. So it’s called gamification now, but if you get set up so you can make it available, like a fun game that people would optimize where things go, that would be pretty cool. I don’t think that’s going to happen at all. The reason being is you can, you can now develop rules to handle that activity and then that can be guided by by people and so we continue to see that develop, but to your point, you know, if we, if we look at some of the things in here Chat GPT as an example, you ask questions like, give me a summary of this or tell me about these things, it provides a pretty interesting summary of things. And, you know, I remember, plugged something in, there are six responses and two are quite good. I mean, they, they’re kind of generic, but two were quite good. One was, okay, probably needed a little more calibration on it. And one was like, This is not right, in terms of the equivalency of, if you have an intern, and you say, give me a summary of these things, do some research, and they’re gonna come back to you four days later, and they’re gonna give you something that probably wasn’t much better than that are most of them won’t. But it still needs to be guided by someone with a bit more wisdom. So that’s the, the general focus of of that like searching for what goes on? What can we do with AI machine learning that can detect patterns in different ways I think of, I think at the risk area, like, since we’re looking at the future, we’re always looking to see, are there correlations dependent, independent variables, and we’ve been limited in the past to limited amounts of data, we’ve put those we make decisions, like you and I were talking about, like ever a stand at the beach, you have ice cream, and was a suntan and umbrellas and stuff. It’s like, how much you’re gonna sell of whatever it’s like, okay, ice cream might be related to temperature, like, what’s the, what’s the temperature? How many people are at the beach could be related to school days, or, you know, whatever, some of those elements that you can see how, hey, when I’m hot, I’m more likely to get ice cream words in your mouth, like to buy it when you’re on vacation, as opposed to during the year. So those are all things that are you have a logical, you know, estimate or hypothesis. And you can set it up against data, you know, like, what’s the what’s the weather forecast for four days. So that’s, that’s probably the most guided areas. But one of the things, you know, you and I talked about, you know, FX risk and some of these other commodity price risk. If we think about big data to tie in big data, it’s like, we’ve lacked data. Now we have massive amounts of data. If we put that data in a place where we could use, you know, AI and machine learning to search, and said, Here’s, here’s a range of datasets, try to find at least correlations between individual items and combination of items, things that we may not think of, you would never say I want to hire 12 interns and say spend entire summer cycling through this whole list of items trying to find correlation. But we should be able to set some of the machines to cycle through that. See if there’s any correlations or indicators, we could test the strength of those. And I wonder if there is there is opportunities there, particularly on the risk area to capture more about risk and find correlations, especially, especially when we think about what are the big challenges for for Treasury protecting the risk assets? One is we tend to diminish those items that have a very significant impact that are very low likelihood, high likelihood, moderate, high likelihood, high risk, we’re everyone’s all over that. But it’s kind of like, you know, we build on floodplains. We build by the ocean, even though there’s rocks on the Hill in Japan that say, Don’t build below this point. Because we forget it because it’s infrequent. I wonder if this is something that can help keep those things top of mind and weight those so that we we think about them more regularly, we avoid the tails. And we minimize that’s a really long tail. It’s so small. Those are some of the things kicking in my mind. I’d love to hear what you think.
Todd Yoder 18:28
Yeah, that’s a lot to unpack. But let me start with. So to your point. And I completely agree with you. So Tim, Treasury from Medtronic, he and I on LinkedIn had tried it a couple of things maybe a month ago or so on chat GPT. He tried a little experiment. And then I tried a little experiment. And mine was about the yen. And let’s just say you were a Japanese pension fund. And you were buying 10 year US Treasuries, and you were hedging the currency exposure of that with hedges, shorter term rolling. All right. So then you get into it’s a very complex question, right? Because then you get into repricing risk and where’s the arbitrage and the curve to optimize? And then if the US starts raising interest rates, all of a sudden, yeah, then all of a sudden economically it doesn’t doesn’t make sense. So then you have Japanese investor selling US Treasuries because it no longer makes financial sense that so I posed a question to chat GPT and it actually gave me it didn’t give me an answer like a human that has spent you know, 15, 20 years in the business and knows but it it gave me four things to think about like use just to your point like a an analyst. You know, it may take them four days to come back with this Same kind of for here are some things to think about. Very high, very general. But it happened in eight seconds, right? So I completely agree with you in on the staffing, as you say, you know, you’re seeing treasurer staffing up, I would, I would agree with you. It’s no longer optional, to have technology awareness and to create an innovative culture. And as George Zen former treasurer of Microsoft, you know, taught me, don’t make it a know-it-all culture, make sure it’s a learn-it-all culture. And so I think it’s even more important that treasures are leaders. Excellent, mine, as you heard yesterday, are excellence, confidence and humility, but understanding the importance of DEI so as they’re making these hires, hire people that look different than the rest of the team, they have a different background and the rest of the team, they have different life experiences and the rest of the team, they may not even have Treasury experience, but be diverse in your hiring. And it has to include the technic technology, you know, so So my oldest daughter, my oldest son, studied in college data science, software engineering, because I think it’s going to be extremely important. But I also told him, you know, you need to study psychology at the same time and, and take a few electives in neuroscience. So I think as you see these treasurer staffing up, those are going to be areas where if they do that, right, and they do it strategically, and they have a good diverse, they’re gonna get a lot because I think one of the most dangerous things to the world right now is groupthink and propaganda, but groupthink is very dangerous.
Craig Jeffery 21:51
Like confirmation bias type thing?
Todd Yoder 21:53
Yeah, yeah.
Craig Jeffery 21:54
Where you hire people who are, it doesn’t have to be across some of those dimensions that you’re talking about could be, we have this view of risk in the same way. And we minimize this tail. And when I call that so rare, when that happened, so that ability to complement each other’s thinking, especially when we’re talking about risk management.
Todd Yoder 22:14
Yeah. And your other point that I really love, as we’re using these tools, how can we take this, the transformers and where we have large amounts of data, and that’s where it can be dangerous, because whatever you train the model on, that data is really critical, right? Because if it’s a bunch of ugly data, it’s a bunch of propaganda data. I mean, you never know what you’re going to get out on the other end. So when you, like you said, if you take that to Treasury and finance, and you start to train it on financial market data, I think you’re right, I think that’s where we have the opportunity to really pick out correlations, relationships, causation, from things that we don’t, I mean, we try to spend as much time as we can, on thinking through these things, but to have a machine that can think it can run millions of iterations and and say, hey, something that we’ve not, you know, it’s not that easy. I have a stand on the beach. And when it rains, I sell umbrellas. And when the sun’s out, I sell sunscreen, it’s, you know, our world. You know, and especially in the financial markets. And, you know, like we talked about yesterday, I think macro economics is where the treasure has the ability to really add value to the business. And like I said, you know, treasures need to see around corners, right. And the only way you see around corners is with the mirrors. And to me the mirrors is to be spent as much time as you can thinking through these these new innovations and the opportunities that it brings to treasury.
Craig Jeffery 24:03
You know, when you were when you were talking there, a couple things came to mind. But one I’ll when I’ll share was this idea of the data and the quality of data that you were talking about. We use BI and we have a what’s called programs that grab data and pull information back. And so one, one thing we’re looking at is, you know, what’s changed with earnings credit rates and all these different curve information that that’s that’s changing is pretty volatile. When we change some of the data that’s coming in and said, Let’s look at it from a year perspective, as opposed to month by month, it pulls beginning of the year, you know, for the Fred database as opposed to like the end of the year. Yeah. And so that fed into one of the charts and we’re looking at it’s like, That can’t be right. Because instead of looking at monthly it was we looked at yearly and it was noticeable, very noticeable to the human eye because it’s those rates have gone up quite a bit. I mean, the Fed has raised rates, ECR rates have gone up, I mean, all these things have changed dramatically. If It had been very stable. I wonder if we would have caught that on a report. Now, of course, we may not have put it on a report if it hadn’t been moving. But that was one of those things where you get the data. But there’s also assumptions like, hey, let’s pull this, and someone else in some other system has a different view of what this data is what they need to show. And so that’s, yeah, that’s just an issue of it. You can’t just like, let the machines run wild, there has to be validation and, and they have to know where that comes from, and then repair and fix those. I know, we can talk about this a really long time. If we’re talking about the future of, of Treasury, there’s these technologies that that you’ve been describing, and expounding on quite well, I want to think about the people, you’ve already talked about some of those things, having the ability to have a team that complements one another, which is really what you’re talking about when you’re talking about some of the diversity of thought, experiences, right? Someone sees resks, like I never saw risks there. But in the example, you know, when we’re talking yesterday, someone said, What are the biggest risks? And it’s like, well, if you talk to us, it’s like what spy balloons have flown over the US? Like that the bigger issue. But when you talk to Europeans, I was just at a conference not too long ago. And it was like all the Europeans like we have war in Europe. And it’s, that’s not what you hear in the US, well, that’s closer to them, a bigger impact. So we’ve got these regional things. And those are the types of things that influence you know, how you look at things like oh, they’re seeing this is a bigger issue than we’re seeing as they probably could care less about actual spy balloons, or, you know, science projects that we shot down with an $800,000 missile, but that’s part of the how do we how do we understand risk? Where do we have our blind spots? Let me give you this story. And maybe we talk about what what’s necessary from employee perspective or mindset. I shared this before. So hopefully those that are listening on the podcast, don’t get mad. I’m not sure if I told you this is the there’s companies that the Zoomers, right, the age cohort, they left and now the manager, director, Assistant Treasurer is having to try to fill that position and they’re doing these very manual functions. Why did the Zoomer leave? They didn’t want to spend time keying in all this information. And you’re saying like, Okay, there’s one part of me that wants to say, you’re just not willing to work hard, right? That’s the negative view towards Zoomers or Millennials or whatever. It’s like, you’re not willing to work hard. But think about it, it’s there actually being very intelligent there. It’s like, I can go somewhere else. And I don’t have the key stuff, what’s the value to me keying stuff and all the time. And I don’t see it’s gonna get relieved in a while. And I can work for at least as much money have a better experience at another firm, either across the street, or I’m still working on my office at home. And to them that makes perfect sense. And you know what, it does make sense. We have the tech to avoid that. And so we might provide staffing for that company and help them automate that. So they can hire someone who, who’s not doing those terrible things. And it’s, it’s not it’s not just by age cohorts, age courts can give us some generalizations. But I think there’s some value of, hey, we’re willing to work hard no matter what it takes. And we’re not willing to put up with bad technology. What are some things you think about in terms of the technology mindset that Treasury has to lean into harder move into more rapidly?
Todd Yoder 28:22
No, you’re exactly right. And that’s an area that I think I would score myself as a as a treasury leader lower on just because these young professionals are extremely intelligent. And like you said, they want to use their intelligence, and I’m big on mentoring. So I have over the years, Julian, I can think of Julian and Michael, Trevor, have just bright young people and Julian is Chinese descent master’s degree, she is a superstar. So eventually, we didn’t have the tools at the time. So she now works for for Fred at Technique, FMC and it was a promotion for her and I helped facilitate, I was so happy to see her move into a role where she can be more analytical, but these tools are definitely going to help a lot with that. On the dangerous side, there’s a list of dangerous things right with this technology with generative AI and the deep fakes so it’s one thing when we get you know, we’ve got I’ve gotten the email on my personal email and on my work email from the CEO. Okay, so what if I get a phone call from him? Now, generative AI can do that. What if I get what if he sets up? Hey, Todd, I want to talk to you video conference real quick. Let’s jump on a zoom. The technology exists. I could have the face to face zoom call with him. It’s scary. Yeah, that’s scary.
Craig Jeffery 29:52
The face to face zoom call with Tom Cruise. Right? It’s like that’s, that’s a great deep fake.
Todd Yoder 29:57
Yeah. And so then if we move to your, and I love your positives. And so I made a quick list of positives. And one of the things is I’m still alive. So 25 years of driving, and 25 years of driving, and I’ve never been in an auto accident. And I tell my kids, you know, it’s because I raced quarter midget race cars when I was four or five years old. And so I learn how to drive. But I can tell you, my wife bought this, this new Escalade thing, and it’s got the driver assist, and so that Driver Assist has saved me, I’m not kidding you at least three times that Driver Assist has stopped that huge truck. And I have six kids. So you know, it has paid for itself. I mean, you can’t put a price on it.
Craig Jeffery 30:48
I owe Mr. Rumble money too for waking me up on a long drive.
Todd Yoder 30:55
So some of the things I’m really excited about are you know, in my past, I’ve done a lot of business, just due to the nature of Fluor in remote countries in emerging markets, where they have different finance ministries, the regulations, the restrictions on banking, are all different. Imagine I have to do so much work on text structure, you know, working with tax working with legal working with the the banks, the onshore the offshore banks, and figuring out, okay, how am I going to run a $5 billion project through this country? How much content has to be on shore with local craft labor, you take like Mongolia, I don’t know what it is now. But it used to be you had to run all in coming out. It had to touch the Mongolian currency through. So those are things you know, restrictions on how deep is the market for that currency? You know, and you and I’ve talked before, I’ve, I think I’ve had the world’s record for trading the largest block of Kuwaiti dinar, which is an extremely illiquid, well, not extremely, but compared to the majors. It’s kind of an illiquid currency. So imagine you could pose this to the machine. And it doesn’t give you all the perfect answers. But if you train it on the right data, it could tell me, Hey, if this is what you’re gonna experience in this country, and you need to watch out for this, this this, and this is insane, because the amount of time and these people that I work with these attorneys and tax professionals, they make serious money, so an hour of their time is very expensive. And on the flip side, I think, you know, it’s going to come back fraud reconciliation, forecasts versus actuals. I heard our friend Bob Stark from kyriba. Talk about the potential for forecasts versus actuals. And to me, that’s rate volume and mix shift plan, how did you do actuals, you know, versus what you plan to do, or year over year, documentation controlling documentations. And then imagine the machine you know, from a controls, policies, procedures, and auditing perspective, you can run certain data through there, and you can get tipped off, hey, Craig, you can place this, you know, $500 million interest rate swap, but you’re in violation of this or this, or you don’t have the board approval, and at that notional amount, you need Board approval, so it really tipped you off to just an extra layer of safety there that I think is will be extremely value.
Craig Jeffery 33:28
I feel we’ve just gotten started, but someone’s actually coming into the door, so we need to end it.
Todd Yoder 33:32
So yeah, we got to head to the swimming pool. Let’s get to them. No, thank you. It’s great to be here and sunny Orlando. It’s beautiful.
Announcer 33:45
You’ve reached the end of another episode of the Treasury Update Podcast. Be sure to follow Strategic Treasurer on LinkedIn. Just search for Strategic Treasurer. This podcast is provided for informational purposes only and statements made by Strategic Treasurer LLC on this podcast are not intended as legal, business, consulting, or tax advice. For more information, visit and bookmark StrategicTreasurer.com.
Subscribe to the Treasury Update Podcast on your favorite app!
Related Resources
Treasury Technology Analyst Report
Researching new treasury and finance technology can be overwhelming. Strategic Treasurer has stepped in to help. Explore our definitive guide to the treasury technology landscape and discover detailed, data-based coverage of each area.
Craig Jeffery and Paul Galloway of Strategic Treasurer discuss the key findings and implications of recent survey data by Strategic Treasurer. They discuss the new advancements of APIs. Are they improving fast enough to keep up with market demands? They touch on the thought process involved in a Treasurer deciding on a payment hub, and the challenges treasury departments face in managing their cash conversion cycle.