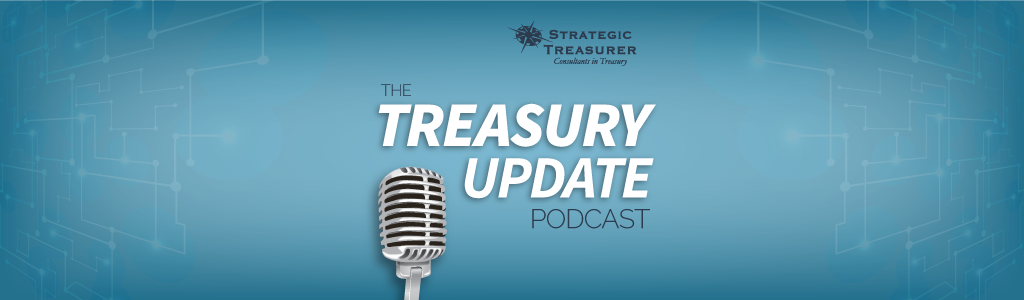
Episode 281
Generative AI in Treasury: A Dialog with Deluxe
In today’s podcast episode, we’ll hear a discussion between Craig Jeffery and Yogaraj Jayaprakasam on the transformative power of generative AI in treasury. They discuss generative AI’s transformative impact on communication, reasoning, and domain-specific applicability, as well as how Deluxe is looking at the adoption of AI.
Speakers:
Craig Jeffery, Managing Partner at Strategic Treasurer
Yogaraj Jayaprakasam, Chief Technology & Digital Officer at Deluxe
Host:
Craig Jeffery, Strategic Treasurer
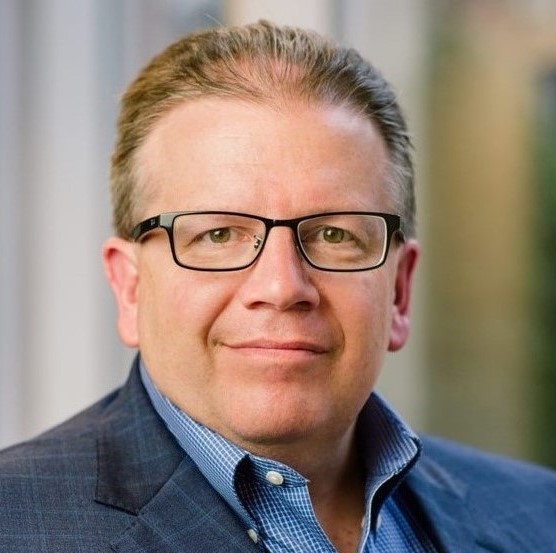
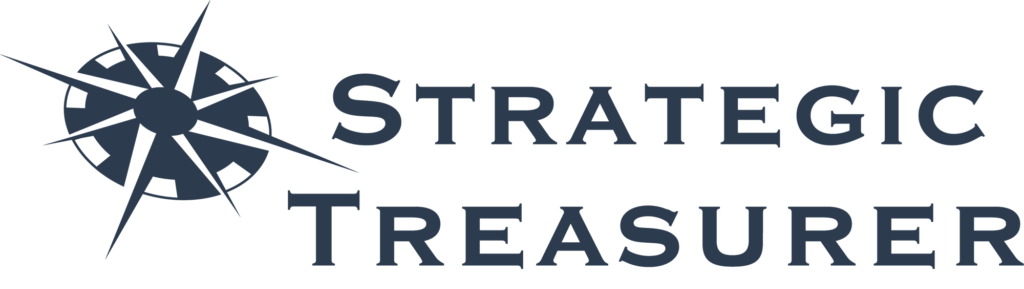
Speaker:
Yogaraj Jayaprakasam, Deluxe
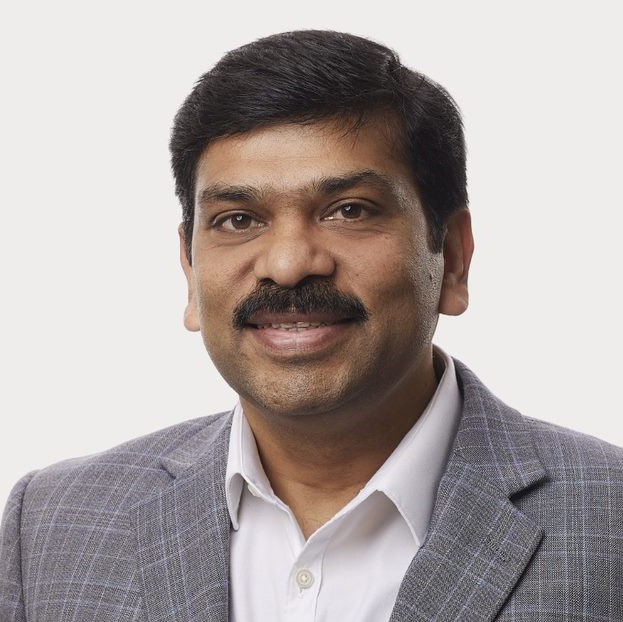
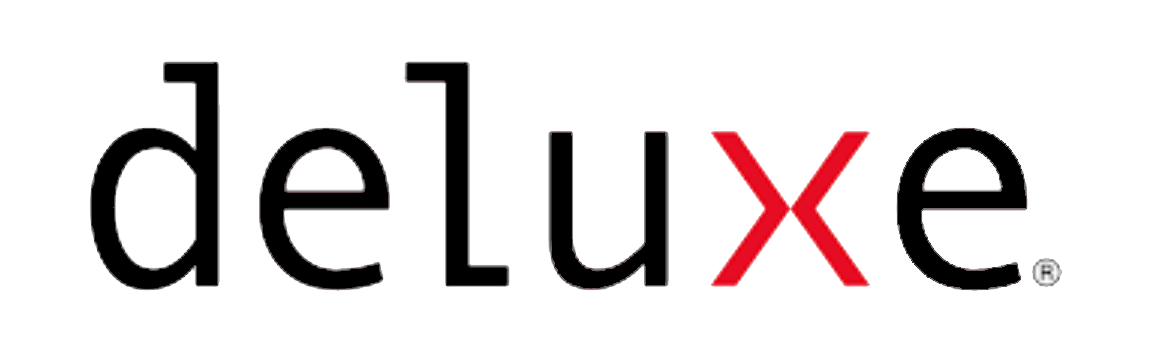
Subscribe to the Treasury Update Podcast on your favorite app!
Episode Transcription - Episode # 281: Generative AI in Treasury: A Dialog with Deluxe
Ep 281 – Generative AI in Treasury: A Dialog with Deluxe transcript
Announcer 00:04
Welcome to the Treasury Update Podcast presented by Strategic Treasurer, your source for interesting treasury news analysis and insights in your car, at the gym or wherever you decide to tune in.
Craig Jeffery 00:19
Welcome to the Treasury Update Podcast. This is Craig Jeffery. And today’s episode is called Generative AI and Treasury: A Dialog with Deluxe. I’m joined by Yogaraj Jayaprakasam. Welcome to the podcast.
Yogaraj Jayaprakasam 00:33
Thank you, Craig, happy to be here.
Craig Jeffery 00:35
I’m excited about today’s conversation, generative AI and Treasury. And you certainly know and many of our audience knows that machine learning AI have been around for for many, many years. But it’s been developing it’s been developing rapidly. And then in 2023, with the release of ChatGPT, it certainly became like publicly available accessible to to everybody with a computer and access to the internet. And this really seems to have stimulated the rate of change. It’s it’s really quick. And maybe the first question is before we talk about generative AI and its position there, maybe you could cover the difference between traditional AI and generative AI maybe help explain how a regular business person can think about this.
Yogaraj Jayaprakasam 01:24
Great question Craig, machine learning, statistical modeling and AI has been a long developing fields. And they have been in use in many, many pockets. But when you think about traditional AI, it is all about in a particular domain and context, using the past data to predict the future. Right, it is so contained in that one particular context. So for take, for example, predict growth based on historic performance of sales. And that is only in the sales domain, you’re applying the data seasonality, various variables, and then finally come until what is going to be for the next two quarters, three quarters. But generative AI, on the other hand, is actually has the power to generate net new content. Also reason with you with that content, because it is you still need the data, you still need the traditional machine learning and all the AI techniques. But using all of the generative AI is now starting to reason with you like a human would do. So that’s the big difference. And that is why it is an inflection point that you’re starting to have the mission help you versus you constantly asking the machine to do certain tasks.
Craig Jeffery 02:45
I started to mention the rate of change has sped up particularly since it became in the public domain. I was going to ask you if you see generative AI as just another step in the journey or is it an inflection point. Clearly you think it’s an inflection point, maybe you could explain what you think the impact generative AI is likely to have.
Yogaraj Jayaprakasam 03:05
So there are many studies and the one particularly that I will call directionally is McKinsey study. And their study shows roughly about 65 plus use cases across many industries. And in the two areas one, the potentially human productivity gain, generate way has really big opportunity to contribute. Their number is somewhere around 60 plus percentage in the next five years time. But on the growth side, you can also reimagine every product and services that are built using computers can be reimagined. You may ask why. Simplest way for any business leader to understand generative AI is there is two things that is changing here. One gen AI solidifies human language as the way to communicate with the computer from using the mouse and screens and selecting various options on the screen to get to an answer. The only other place that you will have done that before is maybe a Google search or Bing search, because you’re just talking to the machine. But there the second biggest revolution there is now you can do it with any system, not just search. But the second is the machine actually can start to reason with you, start to create new content, start to guide you through the process too. That I think is the fascinating breakthrough that we all have to comprehend. What it means is someone who is always used to using computers through a website or a mobile phone. Now you can actually start to interact with the machine without having that interface and clicking multiple browsers and mouse points and things like that. So that is the weight for all of us to think about.
Craig Jeffery 04:50
Maybe you could just give everybody a little bit of background about your your role is a little bit about your career, but most particular about your role at Deluxe.
Yogaraj Jayaprakasam 04:58
Wonderful. My role at Deluxe is the chief technology and digital officer. What it means is all of the technology organizations, including cybersecurity, cloud infrastructure, developing the revenue products, using technology, that’s all under my remit. And we always tell that part of our transformation of becoming Cheque Printing Company to payments and data company is fundamentally changing the role of technology. When we were printing check, technology is the enabler of our business. But when we are doing payments, and data, technology is our business. So it is part of my organization’s responsibility to support my revenue units in achieving all that technology can offer to our customers. Prior to joining Deluxe, I used to work for American Express, I spent about 13 years with AmEx, and most of my time is spent on technology driven transmission, started all the way from modernizing platforms to date, big data driven delivery, including machine learning, then moving into sales, marketing and enterprise platforms to create data and platform construct inside the company. So I’m excited to have this opportunity with the legs, where that is exactly what we are trying to do.
Craig Jeffery 06:20
Thank you so much. On this front of using human language, this aspect of AI, you know, I think of when we run queries on systems, we used to have to know the system language. And then it became, you know, we get used to the using Google or, you know, any of the search engines to ask a question much more normally. And then the the newer version is like, if you’re using chat GPT, you asked the question, say, give me this and put it in this, I’d like a table made up of this information. And it does what you say because it understands the structure. And so that’s, you know, it’s so much more contextual. Even in the early days, it was like a way and significantly better, you know, intern, you ask an intern for something, they come back four days later, it’s missing a lot of information. It’s incomplete. And you use, use generative AI, you ask the stuff and it’s like, in seconds, it comes back. And then you refine the question a bit, and it comes back and it comes back better. It may be missing some information may make some assumptions and may create some fictitious stuff. But it’s it’s way faster and superior to to an intern. It needs guidance, but it’s it’s pretty, pretty powerful. You know, the role of AI in the corporate and treasury, domain financial institution domain. What does this mean to the users of AI and machine learning about the capabilities? What should we be thinking about this in terms of maybe some examples? I’ve got some to share, too. But maybe you could, you could start us with that?
Yogaraj Jayaprakasam 08:01
Absolutely Craig. First, I would love to, I liked what you said about hiring an intern, but only faster, better. The way I articulated this technology to my board is, it is like not hiring any intern. It is like hiring an Ivy League intern. So you got a really smart one with you. They are going to come back with a lot of questions, but a lot of interesting ideas, you still need to put an expert because they don’t have all the experience and nuances of the real world business world. So you still need an expert to validate what they are sharing is real, and you will be able to use it to generate value for the business that you run. But particularly when I look at Treasury Management, I think it will not be an understatement if I say that Treasury Management probably is the last business function, that the world is turning to apply technology and automation. So the good news for us is we have leapfrog opportunity with the generative AI and emergence of generative AI, for example, internationally, you’re managing massive amount of data, payment instructions, invoices that you have to associate that invoice with the right payment, all of those things. But imagine the exceptions that come out of this because end of the day, the success rate for treasury management could be how much of the payment association with invoices, I can get straight through processing. So the humans are not supposed to spend their time as well. Just imagine if you are dealing with lots of exceptions in your process. And now this really smart intern gives you a summary of the exception based on the high rated exception that you need to first enter. So the human time and capital you will minimize to shift that focus to high impact value of, How do you maximize your cash application? How do you maximize your time to cash as well as finding capital improvement for both buyers and sellers? I think that’s fascinating. I even have the dream that this technology combined with the right business expert could create digital treasure. Right? Just imagine you can have a Digital Copilot for treasury management that actually sits alongside with you to perform the function for you.
Craig Jeffery 10:31
I like that the Digital Copilot, you know, some of the things that we certainly see our clients and people in the space using AI, for it’s usually delivered by technology vendors. So we see that in the form of, let’s say, machine learning or pattern detection, for example, can be quality control, it can be detecting fraudulent names, because they’re falling outside the bounds of what typically happens. Those are common. And then, you know, pattern detection, like forecasting. There’s a number of vendors who are who’ve gotten much, much better at forecasting, by running the data, running it through and running multiple models in the environment of COVID was a environment where companies needed multiple models and forecasts, and it’s really hard to continue to run these in Excel. And so it allows this greater extension of running more models more rapidly, you know, in any in any environment, like, yeah, like how you you use the intern, you know, an Ivy League intern, a really fast Ivy League intern, and then pretty soon, Bard or chat GPT is going to be insulted. It’s like, why are you an associate? We’re way faster and more complete. When we’ve been asked questions before, you know, run a forecast, what are the associated variables about what can drive our forecast up or down, there’s the historical information, there might be dependent independent variables, and we’re used to those from history. And you might be able to say, well, temperature will drive, you know, ice cream sales, or the use of home heating fuel, there’s different things that you can see a correlation. But there’s also this aspect of that took time, and you have a limited amount of time, how many things you can look at. And now it’s like time is, that’s not really an issue. You could say, look at 500 more things, and it’ll look at them. And maybe it’s maybe it’s a couple of minutes versus you’d have to have an army of interns with several weeks to do that. And now it can, you can do so much more quickly and maybe see correlations or causation. So and I don’t know what you what you’re seeing too, on the ability to do better reporting this growth of business intelligence tools, much more easy methods of reporting, combined with AI helps ask those questions, get those answers in ways that makes sense really, really rapidly in putting in the hands of, like you said, the digital treasurer.
Yogaraj Jayaprakasam 13:05
No, I absolutely. I think that’s where the power is, right? Because until recently, even organizing data and using visualization tool, all of that used to be very expensive process and getting data right, and how do you then get the actionable insight is the key task. But now Excel sheet more than anything, actually becoming a very powerful tool. Microsoft also introduced Copilot for Excel, which can actually start to act as the digital assistant for your Excel data itself. So I think our ability to ask better questions and get better answers is going to rapidly skyrocket. But I was wondering the other day, Craig, given that getting answer used to be how a subject matter expertise was measured before. And a lot of our jobs used to be Oh, he is or she is my subject matter expert, and I need to retain them and the job. Now no longer getting that information is your problem. I think the problem is now shifting to how well can you articulate the question? Are you asking the right question, so you get the answer that you want and translate that into results? I believe most of humans job is going to be shifting to our way, how do we train our kids to ask the right question, because the education system has to completely change now, it’s no longer about knowing and learning it is about asking the question in my opinion.
Craig Jeffery 14:37
So it’s so it’s we’re back to logic. Now what makes logical sense what’s, what’s reasonable? The first issue I ran into trying to get data historically, for something that’s not doesn’t exist, like if you want interest rates or FX rates, you can get history historical information very easily. We’ve been able to do that for a long time. But if you look at something that maybe is not so common, you know, the search engine doesn’t necessarily find it. So I was like, what are the reserve requirements? What are the reserve requirements, spend, put them in a table, show the percentage and list a source for them and put a table together the US reserve requirements. And I was like, it was 15%. At one point in time, I might forget everybody’s name in the world. But I remember numbers. And I remember it was 15% for a while. And I sent a response back, I said, wasn’t it 15% at one point? And then it comes back with the Oh, sorry. Sorry, we found more information. And then it updated the table, right? It added the table and put that other information in there. So there’s a I don’t know if it’s is it on the human side? Is it you have to know? What’s enough information that that that judgment of what’s enough information, and to use and to filter out some of what’s most important? So is that is that going to be the area of expertise, not necessarily knowing all the data, and I guess this example is something I knew that it just had a gap that will probably be overcome. But is it the the logic and the judgment, that will be the most important skill set?
Yogaraj Jayaprakasam 16:14
I completely agree with you, but I always tell my kids common sense is not so common. You need to apply logic to understand what is the right thing to do here. So as you’re explaining people’s ability to make judgments, based on what they know, and what they are willing to bet on, because you may not know everything, and that’s why you’re going to use this system as well. But the key way that I would think about it is today, the generative AI is trained on what has been already made publicly available as data on the internet. That is the input for that. But the way companies are starting to leverage this is the models that are trained on the public data, you now can take the same model, and give your most sensitive information and retrain it. So it has all the information about the common world. But now you are giving more secretive information that you’re publicly not making it available. So I’m talking about two different things. One is humans judgment, reasoning, logical thinking, ability to question, this all will become more important. But also the models can perform better if you’re training with your own proprietary data that is not easily available anywhere. I love what you said, our ability to make judgment is going to be most critical. But I recently read an article for a CEO, the number one quality for a CEO is your ability to judge because you need to constantly bet on what is going to win next. So now what it probably is going to be the quality for all of us in the age of AI.
Craig Jeffery 17:55
That’s excellent. So another term has been introduced: hallucination, right? Generative AI, creating things that aren’t there like making logical, logical items. I love that. The level of I’ll call it enthusiasm, or maybe even exuberance about using artificial intelligence, I want to ask you about what are what are some reasonable concerns and guidance that you would give to different finance leaders treasures CFOs? What pitfalls need to be avoided. But to set that up a little bit, it wanted to talk through a couple of things. And maybe there’s context for this or not, you know, the use of AI, and machine learning, you know, the domains, like you said, Treasury might be one of the last areas in finance or in companies, I can’t remember exactly what you said. But some of those areas like auditing, for example, they’ve they’ve been using this for a long time, because it can scrape data through financial statements. And instead of having a room of, you know, eight, first or second year, students from business schools are from Ivy League schools or whatever, going through and doing that auditing work. Now they have, you know, one person looking at stuff and then someone managing the process and people reviewing what goes on there, they’re they’re running it through the models. It replaces an army of people doing those functions. Treasury has always been very thinly staffed. AR nobody really funds expense and cost centers very heavily. But to the extent that AR and AP can be more powerfully managed, there’s there’s usually some room there for I’ll say for increase in global automation being able to scale more whether it whether it results in less staff or not, it remains to be seen what the organization looks like. Treasury has always had in almost every organization, this has had many, many tasks that they haven’t got to the should that their significant value. They’ve almost always been very thinly staffed. And so maybe this represents an area where there’s more opportunity, but given this interesting excitement for risk management and Treasury and visibility to everything from counterparty risk to where’s our cash to? What’s our forecast supposed to be? What are the concerns people should have? What what do we need to avoid as well? What do we need to do? What do we need to avoid?
Yogaraj Jayaprakasam 20:11
Great question and great summary as well. Stating what is the reality of treasury worth today and human constrain in that area as well. So the way I would think about approaching this in Treasury is, first wherever you need to train people in treasury management. Because constantly you bring in entry level financial analysts, much younger, financial analysts, is there a way you can apply this technology to even teach them train them, because that’s a lot more easier place to start with, and then start to apply more risk based approach to where you apply this technology. So training is the easiest place to start, then second is co-creating, you’ll still have the main individual doing the job. But then your technology starts to summarize the number of exceptions to the level that they can only look at more high risk exceptions in your systems and things like that. And then you can go all the way up to eventually can you have the technology to help you close the books faster, calculate the liquid positions, faster, capital calculations faster, all of those things. Finance is a highly regulated area, particularly if you’re a publicly traded company even more so. So it will be an iteration for us to get to a point where, oh, I’m going to reduce my audit team by 50 persons. Let’s just give all of this to machine. While the productivity gain is real. I wouldn’t put focus for the companies on how do I cut my people, I would rather put the focus on how can I use their subject matter expertise to generate more value either for your customer or for the company? And also anything that is regulated? Do not apply this technology there right now. So go from low risk to high risk, which is training people, summarizing their work, and getting slowly to assisting with your decision making, not directly applying it to make decision.
Craig Jeffery 22:16
We talked generally about machine learning, and then how does it fit into the space generally, and then some of the pitfalls or concerns and I really do like your training, cocreate. Do these different tasks faster, deeper, better, and moving from low risk to high risk that there’s there’s quite a bit of quite a bit of judgment in their good judgment. But maybe you can share some of the things that about your company, Deluxe. How is Deluxe looking at artificial intelligence, and intelligence? And do you see any distinctions about what you’re doing at Deluxe company level? Maybe anything specifically within the Treasury domain? What are you? What are you looking at?
Yogaraj Jayaprakasam 22:56
Great question. First of all, as many of the audience who knows Deluxe know, data driven marketing is one of our high growth business units. We are dealing with billions of data elements, their source through hundreds of data sources, and where we have been already applying machine learning and artificial intelligence for many, many years already. Because the targeted marketing that we delivered to financial services, it’s all combined with the power of machine learning, but mostly in the marketing area. But now what we are starting to do is take advantage of the technology assets we’ve built to start to source the receivables data, and leveraging more deterministic machine learning to do the matching of the invoice codes and things like that. So that’s all the areas right now we are using machine learning. But when it comes to generate AI, given all the risks that we talked about, we are in the process of rolling out co pilot like capabilities to roughly about 1000 of our employees in different categories. For example, half of that is development engineers. So when you’re modernizing technology code, you no longer have to just do human driven modernization you can apply copilot that actually picks up old code gives you a recommendation on the new codes, etc. So that’s a real use case for us honestly, because someone we all suffer with this old mainframe programmers who are depleting and we still have mainframe systems running. So how do you manage those systems when you’re in your population do not want to work on the mainframes as an example. So I see a lot of potential in that area as well as other copilot like work that summarizes your customer report root cause analysis and things like that. It’s paramount that we are also starting is what the industry would call it as deploying your own private model. So you can take the large language model to deploy it for your within your own company and train it with your own data. So, there is a process called RAG: retrieval-augmented generation that is basically to retrain the model with your own data. We are starting to play with that to see what is the potential there. As I stated for the general listeners, we are applying the same thing, the low risk is help someone to learn something faster, or help someone to do their writing like job faster. And that falls into the copilot like example, as well as for fellow engineers, we are starting to roll out that capability slowly moving into can we summarize the exceptions? Can we summarize the training manual so people can learn about the new system we are building so the end user can benefit from that. And the last stroke would be can we really have our own Deluxe GPT equivalent, that can start to help us with the way we can interact. So that the third step I think is going to be a longer process, the foundational work already started, we first have to believe that what we are doing is one going to be dependable because as you stated, generate a response every time you asked the same question, it can give you a different answer. In finance, you can’t give different answer, you need to be consistent every time you give an answer. So creating all those frameworks to create failproof answer is basically where we are focused on.
Craig Jeffery 26:29
That was really interesting to see what you’re doing in your roadmap for the use of AI. And you know, as you described, Deluxe being a data company, you think back to the history, I can’t remember how old Deluxe is, but it’s well over 100 years old.
Yogaraj Jayaprakasam 26:42
A hundred and eight years to be precise.
Craig Jeffery 26:44
Yeah, people knew it from checks back way long ago. I mean, you still do checks, but that was a, it was, you know, a paper item with ink on it and sent out and now it’s a data company. You know, do you think of it as more of a data company? Or do you think of it more of a analysis company? Because you have data? And now you’re gonna be doing analysis? Is that going to change? Or is it the, the combination of the power of of information and the ability to analyze it and learn from it.
Yogaraj Jayaprakasam 27:14
We think of ourselves as payments and data company payment is our primary focus. And data data is everywhere. So we we want to combine the power of payments and data, both in offering data driven solution as well as offering payments solution. So we recently launched this new platform called Receivables 360 Plus. Fundamentally, we believe that to be a data product more than a payment product, because all we are doing is taking massive amount of payments data, applying additional complex complex machine learning automation, to start to make the life of treasurer and Treasury teams easier and simpler. So we consider ourselves as a payments and data company.
Craig Jeffery 27:57
That’s a great, great explanation. It’s just like when you’re getting, and you move to rideshare as opposed to a cab. And there’s no longer all that the headaches of making the payment on that. It’s it’s now yours. It’s just about the ride and experience. And the actual transfer of value becomes less. And so I mean, that’s somewhat what I would have heard you saying.
Yogaraj Jayaprakasam 28:21
I mean, it doesn’t matter whether we are prominently showing in the value flow for customer. But we want to be frictionlessly showing in every value flow of every customers that we serve.
Craig Jeffery 28:35
Frictionlessly showing I like that. That’s That’s awesome. Yogs, any any final comments or thoughts? This has been a fun conversation.
Yogaraj Jayaprakasam 28:44
Thank you. I love this too. Often when I talk about generative AI or AI as inflection point, people asked me this question, particularly being in payments industry. Oh crypto and blockchain used to be taught like this for many, many years, and they never hit the peak, do you really think this AI is is going to be a game changer. But I fundamentally believe that AI is a game changer. And also the reason crypto and blockchain did not take off the way it’s supposed to. They are created based on decentralized. financing model. Humans love control. So the minute some technology works against what we love, the adoption becomes really difficult. But when it comes to AI, AI works with what we already built, going all the way back to the Greek time, when the library concept was introduced. That’s when humans started collecting information. So starting with that, and then coming to the world of search, because search became the biggest revolution. And that’s why Google is as big as it is today. I think that in that same evolution, this is an inflection point. So it’s not trying to work against the human nature, generative AI works with the human nature. And hence, the integration and it is growth is going to be substantial. We either can continue to fight, but I believe that fight is going to be moot. We just have to get on the wave and start to understand both the opportunity as well, as well as pitfall and contribute to it. So I’m completely honored. I have been playing with this technology for last more than a year or so. And last 10 years of my career, I always associated myself with Gen AI, AI related work and a have patents in that area as well. So I’m really excited for what’s happening here and what it potentially can do to humans too. Of course, there are going to be some downsides. Even autonomous cars came that contributed to some crash. But the statistics shows that that autonomous car contributed crash numbers is much lower than human cast errors in accidents. So there are going to be accidents. But I think the good is outsized compared to the bad that potentially will come with generators.
Craig Jeffery 31:10
Thank you so much, Yogs.
Yogaraj Jayaprakasam 31:11
Thank you, Craig.
Announcer 31:12
You’ve reached the end of another episode of the Treasury Update Podcast. Be sure to follow Strategic Treasurer on LinkedIn. Just search for Strategic Treasurer. This podcast is provided for informational purposes only, and statements made by Strategic Treasurer LLC on this podcast are not intended as legal, business, consulting, or tax advice. For more information, visit and bookmark StrategicTreasurer.com.
Related Resources
Researching new treasury and finance technology can be overwhelming. Strategic Treasurer has stepped in to help. Explore our definitive guide to the treasury technology landscape and discover detailed, data-based coverage of:
- Treasury & Risk Management Systems
- Treasury Aggregators
- Supply Chain Finance & Cash Conversion Cycle Solutions
- Enterprise Liquidity Management
Learn more about these technologies and evaluate some of the top vendors in each industry.