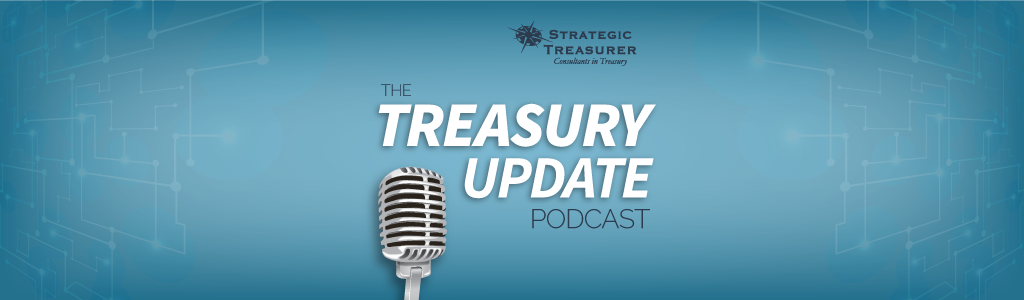
Episode 55
Examining Data – Seismic Shifts in Corporate Treasury Series
This new episode of the Treasury Update Podcast is part of our exciting Seismic Shifts in Corporate Treasury series. From technology and payments innovations to compliance and operational shifts, major changes are occurring in today’s industry, with some already making formative impacts and others still in the “tremor” stage, signaling future hits. Listen in as Strategic Treasurer’s Managing Partner, Craig Jeffery, reveals insights into how regular data, tagged data and big data are major contributors to these seismic events creating tectonic transitions and deep-seated shock waves throughout the treasury industry.
Host:
Meredith Zonsius, Strategic Treasurer
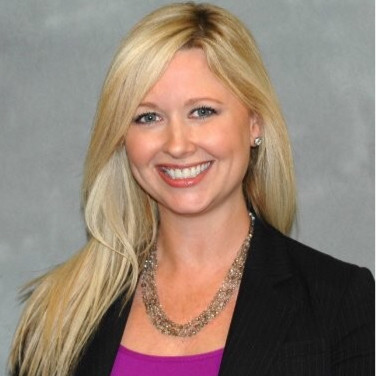
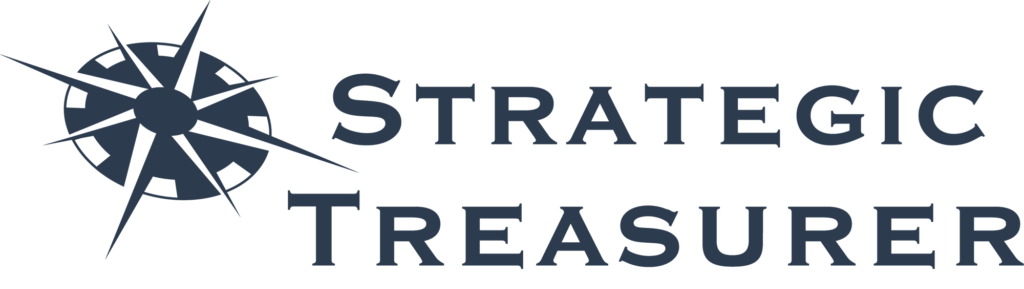
Speaker:
Craig Jeffery, Strategic Treasurer
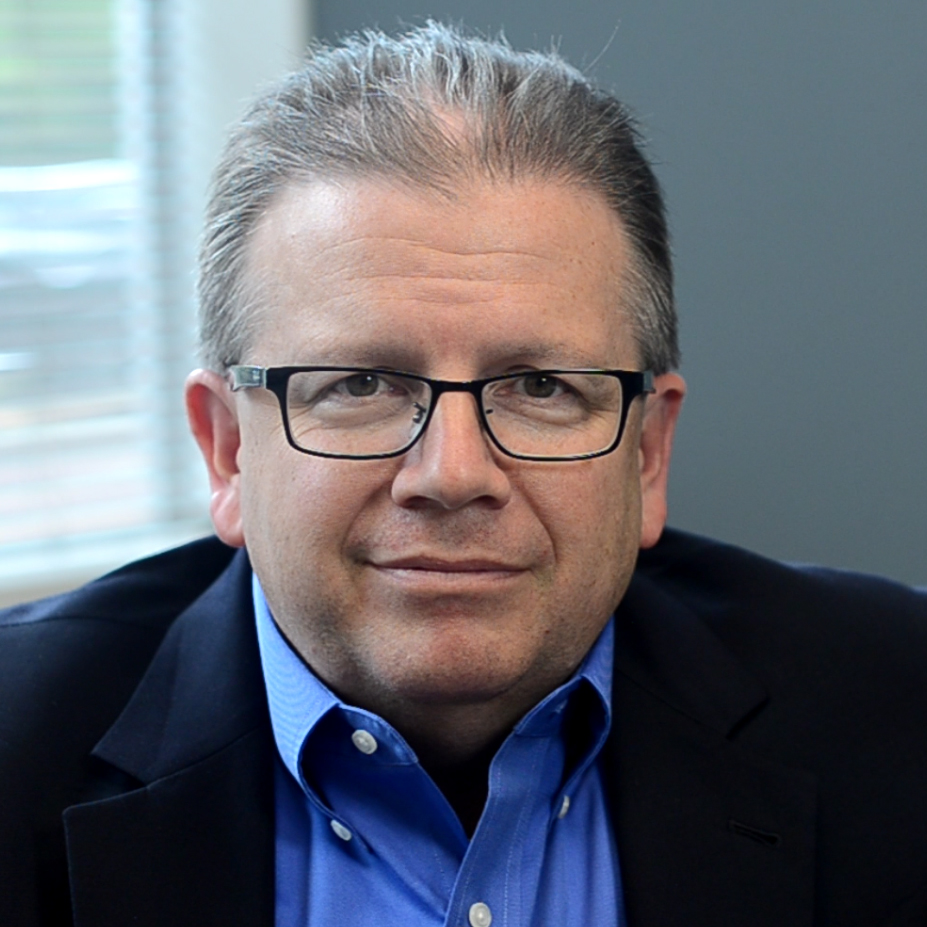
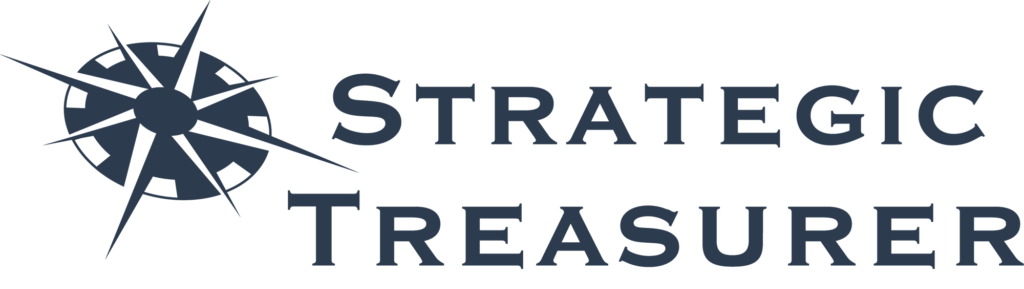
Episode Transcription - Examining Data (Seismic Shifts in Corporate Treasury Series)
INTRO:
Welcome to the Treasury Update Podcast presented by Strategic Treasurer, your source for interesting treasury news, analysis, and insights in your car, at the gym, or wherever you decide to tune in.
This episode of the Treasury Update Podcast as part of a series called Seismic Shifts in Corporate Treasury. From technology and payments to compliance and operations, major changes are occurring in our industry. Some have already made a formative impact and others are still tremor signaling a future hit.
Meredith:
In this episode, we will discuss how regular, tag data, and big data are one of the major contributors to seismic events creating tectonic transitions. Craig Jeffrey is the managing partner of Strategic Treasurer and is also the primary host of the Treasury Update Podcast. Welcome to the Treasury Update Podcast, Craig.
Craig:
Thank you, Meredith. Good to be here.
Meredith:
Great. So data, a major seismic shift is data. I have heard you talk about the significance of changes in data. I suspect many of those who are listening need some background to keep listening to a podcast on data.
Craig:
Oh, hurt me with that. Yes, so I think that’s a fair enough comment. I think there’s a fear of buzzwords and buzz. And so when we talk about data, big data, blah, blah, blah, why do we care about that? I’ll give you an example of buzz. I’ve said this before, eBAM, the electronic bank account management messages, the conversations that you have electronically was a quite a big buzz five or so years ago. Everyone wanted to hear about it. We talked about all the conversations that would take place where you open up accounts, add signers, take signers off. It seemed wonderful and great. And then growth has been slow. It continues, but it has been slow. And so there’s this attitude of I don’t want to be caught by the buzzwords. And so what were, and what are the issues?
It’s easy to say, well not all the banks were set up on it. Some of the banks were printing the messages that came in and they would take that printed document, go to another area and type it in. Well, sure that was some of it. But the majority of it was that companies who are managing their bank accounts had this view that their management of them was very excellent. It was leading and the problem was most of the organizations, the vast majority have their bank account or had their bank account data in a combination of paper, some excel spreadsheets, maybe some different databases, but it was neither accurate nor current. And since it wasn’t all electronic and it wasn’t accurate, it wasn’t current, you can’t necessarily just automate and start sending these messages have that built into the process and so that was the whole transition became a buzz.
It’s a great process to send and have these conversations electronically, know your customers and have accounts. All those activities are vital and they’re painful, but you have to be automated to make that happen. Now that’s a key element for why you can have buzz. The area’s not ready for it. But here’s some additional contexts I think that will help us imagine and understand why data is a very strong contributor to the changes. Or as you say, tectonic shifts already and if not in your organization will become a massive contributor. So let’s just think about growth for a second. Data is increasing at the rate of 40% per year. That doubles every two years. Massive quantities of data, not only on the Internet but accessible. That’s massive, impact. Capacity leads to richer data. eBAM showed that we need to have the data stored electronically and have systems that manage it.
But think about the capacity when we have richer and better data and we can send it around. We moved from delimited data to tag data, and this is where people will turn off. Delimited data is, “I know where the value is in a file based on the position of the record.” So it’s delimited by a comma. So after the third comma is the bank account number, after the seventh is the transaction amount or whatever it is. It’s delimited by a pipe, a filter, a tab, whatever it is, and it was created that way because data was too expensive to ship. The transmission was too costly, so they had to compress it. Well, it’s delimited. Now it’s tagged data. In other words, the seventh record that determined the transaction amount, for example, is now it has transaction amount wrapped around it.
It’s tagged. The system knows that the 5,000 is 5,000 USD and it’s a transaction amount and it knows it because you know as an XML file is as a tagged record, it has its descriptor going along with it. It doesn’t need positional determination. It has that with it. And that makes a big difference because it’s stronger and more resilient. What I mean by it stronger, well, it’s easier to process. It’s easier to adapt. It’s easier to change. If you start inserting more information in a tagged file like XML and you don’t need it, your system just overlooks it. If you stick another field and record six and push what was in records seven to record eight now it’s no longer a transaction amount. It’s thinking it’s description and so it can break the process. So what that means is that these, the tag records, the use of XML for example, allows for a much more resilient and robust process and we can do more with it.
Now a file that comes in that treasury cares about or that AR cares about for posting now that can possibly be used by both areas, or also reconciliation, because you can include more information that payload and it allows you to reconcile, to post, to auto assign and auto apply, assigned to the receivable entity customer or down to the individual invoice. So we don’t break up process when we add more data and it’s more resilient. It allows for smarter processes, it saves us on costs for data, and so richer data allows for smarter processes. I’ll keep going on a couple other elements there because I’m on a roll. So the other element about data and data is just a means to an end. So I’m not that excited about it, but data is a key element of the modern treasury conceptual architecture stack.
So the architectural stack what are the components in there, and we use the term conceptual so that the true IT people don’t get too concerned. But from a conceptual standpoint, we think about data, connectivity, systems, reporting and analytics. It’s sometimes reporting and analytics merge together. Same thing with systems, but thinking about those conceptual layers in the architectural stack, data is vital and more data is more important than ever before. And there’s a lot of changes that are going on there. And I know we’re going to get into that a little bit later, but that at least provides enough elements to get started in terms of an introduction to data as one of the key elements of this tectonic shift or the seismic shifts in treasury. So this is a key area. It’s one of the key elements of the tech stack and it is definitely impacting what we’re doing now and what we’re going to be doing soon.
Meredith:
Excellent, growth in capacity allow us to have enriched and smarter data. What other changes are providing an environment for data to occupy a front-of-mind position for treasurers?
Craig:
Here’s a few items that you and others probably know, but let me do some additional synthesizing and pull them together. So what’s happening? So computing power. We know the computing power has grown radically, there’s even some principles about how quickly at doubles and of the period of time it doubles. It’s been consistent in terms of growing in speed and capacity, getting far more affordable. So what’s in your pocket is more than a supercomputer not too many decades ago and it costs but a fraction. That’s one. Second is connectivity. The cost to transmit data declines consistently. How many people are watching, streaming one of those movie service subscriptions at their home? You might have two or three going on at once. No problem with capacity and yet you’re using hundreds of [megabytes] or [gigabytes] a day without a problem, without even a slowdown that’s at the home use.
But connectivity used to cost a fortune and that compressed data, it made it harder to take advantage of the electronic world. But as we’ve moved from physical devices to electronic and the electronic has gotten far faster and far more affordable, connectivity’s made significant progress in that allows us to pass more data more quickly, more affordably. And third here is analytical tools explode. You know these business intelligence tools, second generation business intelligence tools that have in-memory calculations, they support the self-service and self-querying self-discovery. This changes the approach for how we look at analysis from this linear approach. And I’ll just describe that briefly. If you’re looking to say, I need to do this reporting, I need to solve for whatever this particular issue is, why do we have this problem, or what has happened to, why is our forecast off? Is it due to macro-economic conditions?
What are those? How am I going to analyze that is it due to housing starts? Is it due to increase in interest rates or GDP, whatever those elements are, or I’m just trying to do reporting. The typical approach has been I need to know what I want at the end, so I think of the end state first and then I say, where is that data? What format is it in? How do I get it out and where do I load it? Do I preposition and put totals in? Right. I put it in some kind of reporting cube that has sub totaling in. So my responsiveness to the system will be faster. And that’s great. I mean it’s, that was what was great and was what was modern. But what happens when someone looks at their reports the first time, as soon as they look at their reports, they have three more questions.
And then what happens? They say to the person who gathered this data, who took this linear process? No, go back down the mountain, roll that stone up again. Figure out, cause I just asked you a new question. You need to go find out where that new data is, what format is it in, how do I normalize it and get it into my cube? How do I run my reports off of that? And as soon as they see that, which is two, three, five weeks later they ask another set of questions and it keeps going back and forth in that process. And so that’s, you know those who like ancient literature, you know Sisyphus always rolled the rock up the hill and then it would, you’d have to keep doing that for eternity. this constant redoing this linear approach that has to be recursive in that way.
And the analytical querying tools, the explosion of them is that in-memory calculations and the ability to connect to different data means when I have that next question, I just attach to more data or I pull in data that exists that I haven’t been using. And now I know my next answer and then I know my next answer and when I need something else, I attach to another set of data, whether it’s referential data, static data calibration or rating data, structural data, geographic data, all those elements. Now I have some ways of linking them together to create a faster and better method of looking at it. And so those things coming together make a very significant difference. I want to summarize that piece up cause we’re talking about computing connectivity, analytical tools. The transition that is happening with this is that we’ve moved from a time of very expensive data, a lack of data to, it’s increasingly affordable data too. We will have so much data that we have to use automation to make sense of it. It’s one thing to say, well I can’t answer that because we don’t have the data. The data’s not good. Now we’ll have more and more data. So our responsibilities shift greatly because now we’ll have massive quantities of data which can overwhelm us if we don’t use the tools the proper way.
Meredith:
What is the treasurer to do to take advantage of better computing connectivity and analytics analytical tools?
Craig:
I’ll start with this. I think a couple ideas besides planning and thinking through it. I think that’s pretty obvious, but I think one is how do you think through it and I think first is consider the modern treasury technology stack in light of these systematic changes. And you’re going to ask a lot of questions. I’ll just go through a couple. So what formats will you select? Will you use, are you gonna use the older style formats that are delimited? That’s quicker. but does that set you up for the future? When do you make the decision? Is it all what makes the most sense for the next month, the next year or the next two decades? So it impacts the format you select, it impacts where you put the data. Do you put the data in a directory and a data lake? What I mean by that is if you have several systems, you have something that helps you handle risk.
Maybe you have a treasury management system, you’ve got two or three different bank platforms. Maybe you have something with supply chain, finance, whatever the number of systems you use. You have a lot of data that you need to analyze that you need to report on and not all of it sits in the system. One system that has a reporting overlay, even if it’s a nice BI overlay (business intelligence tool overlay), you still need to make sure that you can run the data in the report. So you have to get at data in different places. So yeah, we moved stuff to the cloud. We still need to access that. You may have to access it differently, you may need to pull some of that data on site or maybe you pull it to your corporate cloud data storage area so that now you can use your tools to pull data from your TMS to pull structural data.
You have to pull analysis that you’ve done in Excel, other databases, information you’ve extracted from the ERP, from other third-party sources. Now you have the tool of what is necessary. So just thinking about formats and where you put the data is a is an access question. That’s only one part of the tech stack. I think the second thing that, well the second thing I wanted to talk about was thinking through 5 C are flexibility, insight, visibility, efficiency and control. In concert with the conceptual technology stack, we have data connectivity systems, reporting, analytics, but with the concepts of flexibility, insight, visibility, efficiency and control. What do I need to do and I’ll just touch on a couple of those. For control, I have data, I have lots of data, I can access it. I still need to protect data. I still need to use the concept of least privilege.
In a paper-based world, least privilege was not really thought about so much because it was only stored in file cabinets and you lock the file cabinet. It’s not copied, it’s not moveable as much, but the principle of least privilege means that I have to control who can access payments and what goes on. The level of thinking that we have has to expand there in terms of how we control it so that it’s efficient so that we can do the type of reporting that it’s anonymized in a sense that confidential data isn’t lost but that, so we can still do our analysis. And that’s very different from one, we don’t have access to data insight this idea of doing analysis, understanding the connections between one area and another one activity and other multiple dependent variables and independent variables. How they interact is one other aspect of flexibility, insight, visibility.
What do I need to do to achieve better insight and what insight do I need to get answers to questions that I don’t know yet. So how does my data need to be and my access to that data and my ability to manage, analyze different situations. If I don’t know what questions I’m going to be asked in the future. And that’s not a mind game. It’s how do you set up a flexible structure and not only mentally flexible but systematically flexible so that I can know that I’m going to have more questions than I know what they are today. I don’t want to have to go back to this linear approach all the time. I want to be able to expand what I have and continue to just make it additive so I put more data in and then I linked to it.
That is something that is a massive opportunity for Treasury because Treasury looks forward, they have to look and see what’s around the corner, not just what’s up to the corner, but what’s around the corner, what’s in the future, what can happen from a macroeconomic level, from a company sales perspective, they have to be able to run models, analyze, look at different scenarios and attach and calibrate risk levels with third party figures, rating agency information and different analytics. That’s the second. And then just to keep it so it’s not too long. I think the third area is setting some goals. And this is just being more proper in this one is formalize your conceptual technology stack. What does that look like? Each layer data, what do I need, what does it look like? And not just saying, oh I need bank data.
I need to know what my investment data is, my debt, who my counterparties are. But think through all the data that you need for analysis, managing counterparty risk, looking at exposures, helping treasury respond to the questions that it should be asking. And that will probably be asked of the group. So formalizing each of those layers data connectivity systems, reporting, analytics as well as the other elements that are part of that stack that run along the side, robotic process automation and then AI / machine learning, some of the areas that we can use to leverage all of our activities and processes. So that’s one formalized conceptual technology stack in terms of what it needs to look like and how it will live in your organization. And then in depth about data is identify how you’ll organize data. How are you going to do your reporting within an individual system, within a bank platform, within your card services company?
Are you going to put stuff in a data lake or a data directory? How are you going to run that and run those models? Is it going to be self service oriented or dumb reports? Reports that just get sent. And maybe that’s not a good term, but just reports that aren’t it’s like, okay, I create it and then it’s not changeable. It’s not alterable. But recognizing that there’s more along those ways. And then I think the third piece under goals is make sure at least some people are experimenting with moving and validating data. They’re experimenting with robotic process automation, using the tools, gaining facility with them and achieving time-savings. So they need to be going through that process. This is get a few pilots going with robotic process automation. I don’t care how, but make sure you do it.
Make sure you’re getting people to learn on this. This is not a buzzword. The tools exist. I mean, unless you’re in a paper based environment, robotic process automation, these bots will help different elements. You can start small and continue to expand and allows you to cheat your way to straight through processing. And I think the last item here on goals is to say this, and this is a little bit of a soapbox item for me, is your default position on data should be to use better formats not the older formats. Use XML, not delimited, whenever possible. And the whenever possible needs to be not a quick dismissal of and say, “Yup, we’re going to use MT940, we’re going to use BAI2.” But don’t quickly dismiss that look to use the better form as anytime you make a change move to better formats.
Meredith:
Craig, are there downsides to these changes?
Craig:
Changes to growth of data, the access of data and speed? Yeah, there’s quite a few and it’s, what’s an advantage for the corporation, the organizations to leverage the data are, I guess one thing, it makes it easier to steal stuff you don’t have, all your data is not stored on paper. In a cabinet where someone has to break into a building in a location and get at it. It can be accessible from anywhere I can. I could be sitting in some state in the US and hack into something in another country, or I can be in another country and hack into another country’s data, move funds and make it easier to steal. That’s one big one. And you can see the rise of criminality the rise of their payoff on the crime, and this means that there’s a downside, which means some of those elements about a, how do we protect data are the 12 security principles we use to protect data has to be incorporated.
And it has changed because of how data has changed, and we are slow to react. We are so slow to react to these major shifts that have occurred with data, with connectivity. The point that even if you have data that’s encrypted, we have to think long-term, and we have encrypted data and someone has lifted that data out of organizations like they have. And nation-states may steal this data, they can’t decrypt it now. It’s too complex. It’ll take too many hundreds of thousands of years to run, but they don’t have to decrypt it right now. They can keep it and eventually computing power will catch up. Quantum computing will allow them to scale up and then crack those old encryption things at some point. So protection of data is not enough to encrypt it by today’s standard but protect it, encrypt it, put multiple layers of protecting those because what works today, what’s a good defense today won’t necessarily be a defense tomorrow.
So protect your data at all times. Don’t let someone lift it now and then maybe they crack it 10 years later, you know when the impact comes later. And then I think the other downside to these changes is that not everyone wants to keep up or keeps up with the changes in terms of understanding what we need to do with data and how to use the tools to analyze data, how to use the tools to manage exceptions and provide alerts to use and program the tools such as robotic process automation, some of the bots that you can program that help shortcut and make the move to straight through processing to increasingly move to straight through processing, reduce the number of rejects that reduce the number of errors or defects in a process. So the idea that not everyone can keep up with the changes or wants to keep up with the changes is definitely a downside because that means people are going to be to some extent left behind.
Now if you’re making sure your organization is testing things, it provides a longer adoption cycle. You can’t all of a sudden say, I’m moving paper around one day. And then the next thing is I’m doing everything with a wireless device. The size of a deck of cards that I carry around in my pocket that has massive computing power. People need time to change in the amount of time they need to change can vary. And so the sooner we start our organizations moving to the next process, piloting and learning, the more people that will be brought along the way and the easier it will be to adapt to that change. So I think that’s a downside that can be handled. And I think it’s, people going to get scared. Robots going to take my job. But if you don’t change what you’re doing, if you don’t continually reinvent how things are done as an individual, you’re going to run into problems.
And if your organization doesn’t do it, your organization will be replaced. Right? We’re not making buggy whips anymore, right? That doesn’t exist. Or maybe they are making them somewhere, but there’s not as many organizations making things for how the world was. It’s what needs to change and we need to make sure we’re advancing the efficiency that controls how we view things and making sure the organization is flexible and adaptable.
Meredith:
Any final thoughts?
Craig:
I would just summarize a couple, this idea of the concept of their Seismic Shifts in Corporate Treasury. There are so many changes taking place from data, from risks, from macroeconomics, that these are really key times to make sure you’re, you’re listening to people that make a lot of sense, that are thinking about the future with their, their heads not stuck in the clouds, but they’re thinking far enough along the way and there’s practical steps to get there. And so I, I’m really excited about this series talking with a bunch of people on a number of topics that help us prepare for this change that help the industry move forward, cause a more efficient treasury is better controlled, it provides a better defense against criminals and makes organizations more efficient. And by making it more efficient, it makes all of our organizations better and that’s very helpful and so I really appreciate the time to talk about this.
Meredith:
All right, excellent. Thanks Craig. This concludes the episode of this special podcast series Seismic Shifts in Treasury – Examining Data.
OUTRO:
You’ve reached the end of another episode of the Treasury Update Podcast. Be sure to follow the Strategic Treasurer on Linkedin. Just search for Strategic Treasurer.
This podcast is provided for informational purposes only and statements made by Strategic Treasurer LLC on this podcast are not intended as legal, business, consulting, or tax advice. For more information, visit and bookmark strategictreasurer.com.
Related Resources
#TreasuryFAQ – YouTube Playlist
Check out our YouTube playlist covering many frequently asked questions in treasury!
Becoming a Treasurer – A Treasury Update Podcast Series
This series within The Treasury Update Podcast explores questions around being a successful treasurer. Topics discussed include preparation, what needs to be measured, effective communication, development of a team, and acquirement of resources needed.