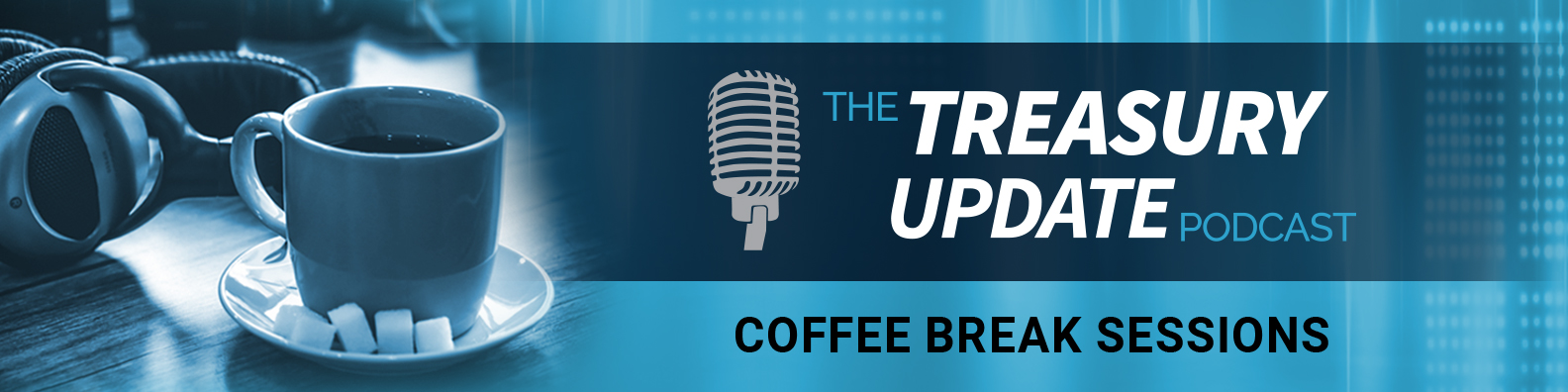
Session 92
Coffee Break Session: What Is Generative AI?
In this episode, Craig Jeffery shares how generative AI can enhance data analysis and decision-making in treasury operations. Is using generative AI considered “cheating”? Is it safe? Can generative AI really help you make accurate decisions? Listen in to learn more.
Host:
Jonathan Jeffery, Strategic Treasurer
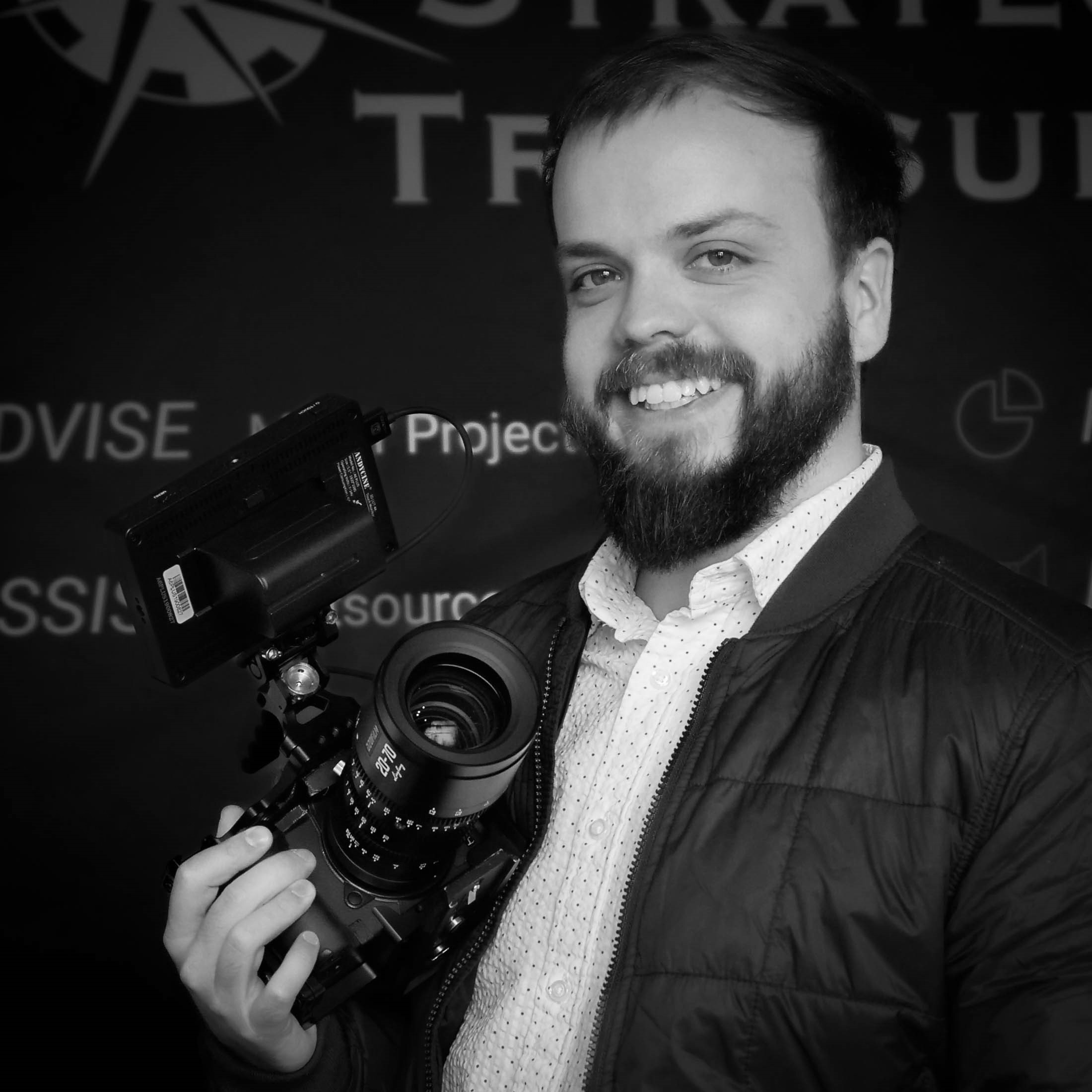
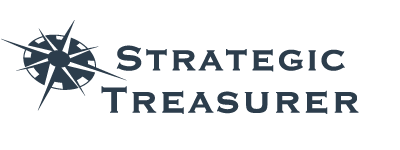
Speaker:
Craig Jeffery, Strategic Treasurer
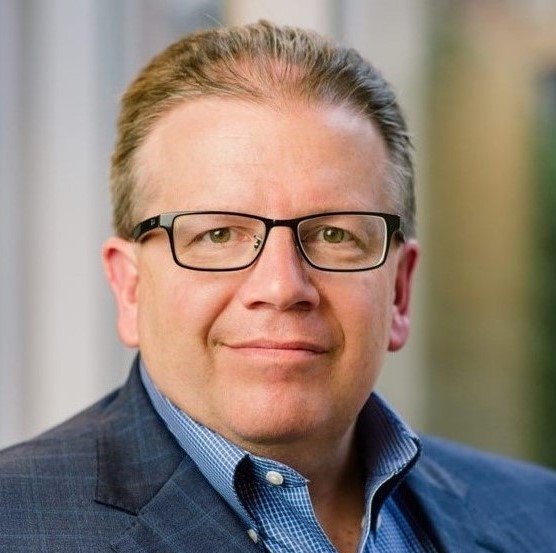
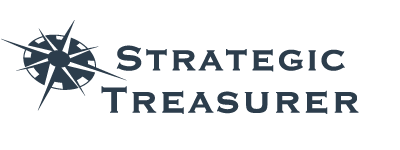
Episode Transcription - (Coffee Break Session Series) - Episode 92 - What Is Generative AI?
Jonathan Jeffery 00:02
Welcome to the Treasury Update Podcast Coffee Break sessions presented by Strategic Treasurer, the show where we cover foundational topics and core treasury issues in about the same amount of time it takes you to drink your cup of coffee. I’ll be your host, Jonathan, media production specialist here at Strategic Treasurer. So sit back, relax and enjoy the show. We are here with Craig Jeffery for another Coffee Break Session. We’re continuing our series on technology and data. Today we’re going to talk about generative AI. Welcome to the show, Craig.
Craig Jeffery 00:37
It’s good to be here, Jon.
Jonathan Jeffery 00:38
So Craig, how does generative AI work?
Craig Jeffery 00:41
Well, I’m not sure I can answer exactly how it works. But what is generative AI? It’s artificial intelligence. It’s using the computer programs, languages and routines to generate new material to create and synthesize different data elements and put it together using patterns and methodologies that people would use just way faster. I’m certainly not a artificial intelligence or generative AI expert. What I do know is that you create these models and systems for learning. And you have to train the system and the training. As you’ve seen with Chat GPT, there, they have a massive scale that they’re doing training, helping the system to work and get better and learn how things operate, you know, so that it can answer questions properly and pull data that’s relevant. It makes all kinds of mistakes, it makes math problem mistakes, it gathers the wrong type of data. And so we have to be careful about especially as we’re learning, just like we have to be careful with any person, we have to be careful as the systems are learning that we don’t rely or overly rely on what’s occurring. But the training aspect is maybe people can think back to not too many years ago, when there was computers playing computers for chess. And one of the models was you have the load all the historical games that were played in tournaments, into the systems that had this giant compendium, all the games are played by humans, to learn from that and to use that to be smarter. And so when computers were playing computers, that was one of the ways they they advanced and did really well. But as time went on, they were able to build systems that would learn the rules and operate within the rules. And it was, it’s a pretty interesting story. I know, we’ve covered some of this on one of the other podcasts. And we’ve talked about before, as they set a system to play itself. So they had the computer playing itself. And it would learn and follow the rules. And it would play it played millions of games and a nine hour period. And that nine hour period, it learned how to play chess, and it got to the point where it destroyed the other chess computers that existed that had the full compendium of all the Grandmaster games that were ever recorded. And it was determined that in about four and a half hours, it had surpassed that, because it learned it played and and saw it and was able to evaluate each of the games. And then it can learn through the process and get smarter in nine hours. While you can’t play million games in your life, I don’t think that would be possible for a human to play a million games. But let alone play multiple millions of games in nine hours and learn rapidly. And so the ability for those systems to learn and to learn rapidly, is a significant advantage, especially as we can use those to think more and support what we want to do. So generative AI works by making connections and by learning through this massive scale, far different from just search, right? It took a long time to make search smart and serve up links that you have to go and research and read. Well AI now has the ability to pull the different searching together not only categorize and prioritize those, but summarize multiple points of information. You can even ask it in certain ways where it’ll provide you with the sources that matter why it rates something higher than another. And so you have a way of scaling what it’s biases. In that process.
Jonathan Jeffery 04:08
The AI playing chess, if they were playing against somebody who had no idea that they were using AI to make these decisions that a human would get crushed. And they would say that the person is cheating by using AI. Right?
Craig Jeffery 04:22
If it was defined as we’re just playing each other. Yeah, that would be cheating. Because you’re using you’re using some other tool. It’s like, Oh, I’ve got two grandmasters sitting next to me or telling me what to do. It’s like in a chess tournaments like you against me, not you against me, augmented are supported by other tools to help assess the situation.
Jonathan Jeffery 04:45
So in treasury, if they’re starting to use AI to make these decisions and forecast the companies that are doing that are going to do so much better. You’ll have to use AI to even keep up in the future.
Craig Jeffery 04:56
Yes, I mean, that’s that’s the way it is with tools and technology over time. I was like, Okay, I need to dig a hole, okay, I’m not gonna scratch it out with my hands. So I got a stick, and then I get a shovel. And that, you know, makes it so I can do 10 times more digging, and then I get a bobcat. Now I can do way more than we get back hoes and giant earthmoving equipment. The better the tool, the more effective that tool, the more you’re leveraging what your capabilities are. And that’s, that’s really powerful. So same thing with digging. It’s not, it’d be unfair, we’re saying we’re having a contest, a digging contest. And we’re both expecting to use shovels and you come on with a giant backhoe. But there’s no restrictions on what are you going to use when you need to dig a hole, we’re going to use what’s most effective at digging a hole. If I can use like before I trade something with FX and this has been around for a while. I see what my exposures are. I know what the risk level is, if I can use the tool on a pre trade basis before I actually spend money that says Here are six combinations. Here’s what it’s going to cost, what the cost effectiveness is of these trades, do these four trades, not the six, it’s going to cost me less money because I trade less. And perhaps I get a better rate or it’s more effective, I have now become more efficient. And that is far more powerful. Most people aren’t using tools. Why wouldn’t you use tools that makes sense? This is this is our whole path, use use more tools, use them effectively improve the performance.
Jonathan Jeffery 06:24
And stay on top of what’s new. Learn about the new tools. Alright, we got to keep moving because this is going to be a very long Coffee Break Session. Can you share a couple of examples of successful implementation of generative AI in treasury operations?
Craig Jeffery 06:38
Yeah, I don’t know, there’s a whole lot of generative AI happening in Treasury right now. And I could be wrong. And so if anybody’s listening, you know, like, we’ve got tons of generative AI that we’re using in Treasury, besides helping to write letters like maybe bank letters or filling out KYC stuff. I’d like to know if it’s if it’s if it’s more than that. But certainly for from a machine learning, or pattern detection approach. I guess I would look at it this way, John, and you tell me if this is too much for the particular podcast, if I look at past information, current activities, maybe to some some view to the future, I want more options to look at the future, perhaps farther into the future, or look at different type of arrangements, pattern detection, and machine learning can fit into each of these. Let me give you some examples. If I look in the past, what are some historical trends? From my cash flow? For example? Oh, is there a cycle to it is a monthly cycle quarterly cycle? Are there different activities that occur? Oh, that’s interesting. Now I can see what occurs that helps me plan for the future. That’s leveraging the power of technology, the power of pattern detection, and mapping and seeing things that are correlated that helps me do something that helps me plan for my borrowing needs, determine how much money I can invest or what that I can pay back. On another pattern detection, plays. systems can be really good at detecting what’s standard and what’s not standard, what falls outside of the normal range. This idea of anomalous activities, things that are not normal, they’re not in the regular range. The anomalies are things that are different is great for fraud, hate, these activities occur too closely together, changing address request for withdrawal, all occurred with an extremely short period of time, that probably needs a human to look at it. Pattern detection of seeing what goes on can also help detect things like errors, not just fraud, but errors. And so you can catch things before they get too far down the process to say, this is wrong, this order entry is wrong. Now we can fix it, that we don’t have an error. Since we don’t have error, we don’t have a defect that needs to be repaired. So those are some of the things that are current that also touch on the current for future, the patterns can be projected into the future for forecasts. And that’s been done in Treasury, I wouldn’t say regulate but by multiple companies, a number of companies. And they’re mostly leveraging technology provided by some of the Treasury system providers to take that pattern that they see in the past, and roll that forward to the future. The other area that I don’t see so much is in terms of using AI or generative AI. In the future, it tends to be done more manually now is looking at scenarios. People may plug in scenarios where interest rate changes within a certain band or foreign exchange currency pair shift and combination. Those can be put together and run run different models or run some some type of simulation across that to see the range of what happens and have expected outcomes. So we’ve we know how to run those. But there’s certainly capabilities of doing that or the possibility of using generative AI to run through all kinds of scenarios not not just one Is that you plug in and run, but to look into examine other factors and say, find other things and run those in a model and spot anything that may be interesting. And who knows that could come up with geopolitical issues, trade, weather, anything I mean, who knows what those could bring up because it good now cycle through lots of things that people may not think of. And there may be some type of correlation to cash flow risk, counterparty exposure or something else.
Jonathan Jeffery 10:30
Yeah, this can be interesting for a podcast in the future on our regular show. If we hear any stories this year at AFP of how someone used AI to streamline something or make their job easier, specific examples, we could bring that back in, in a show. We have a flash survey coming on this soon, don’t worry.
Craig Jeffery 10:50
We do. We’ve done these rapid researcher flash surveys from time to time on different topics. And we we did a poll during the month of July, what topics would you like to hear about soon. And the number one issue was generative AI, artificial intelligence. And so that’s going to be our rapid researcher flasher that’ll be coming out fairly soon. So I’m looking forward to seeing in more depth what people are thinking, what they’re doing, what they’re concerned about, and what they’re planning.
Jonathan Jeffery 11:20
Cool. And after that survey goes live, there’ll be a link in the show notes where you can go and find some information on that if you’re listening later. Thanks, Craig, for joining me on this longer Coffee Break Session. This was fun to our listeners, remember to tune back in every first and third Thursday of the month, and we’ll get a new Coffee Break Session out for you. Hopefully you guys liked these technology and data episodes. Thanks.
Craig Jeffery 11:44
Maybe you can run this through an AI program and say, say all the good content that’s there, but shorten it up by 50%, because Craig was pretty wordy. And that would be if you could do that and you could put it as a parallel one. That’d be pretty interesting.
Jonathan Jeffery 11:59
That’s what I’m looking forward to, AI just put in a video and say make this good, and it just does it.
Craig Jeffery 12:05
Make this good.
Jonathan Jeffery 12:06
My job will be obsolete. But hey, I’ll just manage the AI. Alright, well thank you guys for joining us, have a good one.
Announcer 12:18
This podcast is provided for informational purposes only and statements made by Strategic Treasurer LLC on this podcast are not intended as legal, business, consulting, or tax advice. For more information, visit and bookmark StrategicTreasurer.com.
- Treasury & Risk Management Systems
- Treasury Aggregators
- Supply Chain Finance & Cash Conversion Cycle
- Enterprise Liquidity Management
Learn more about these technologies and evaluate some of the top vendors in each industry.