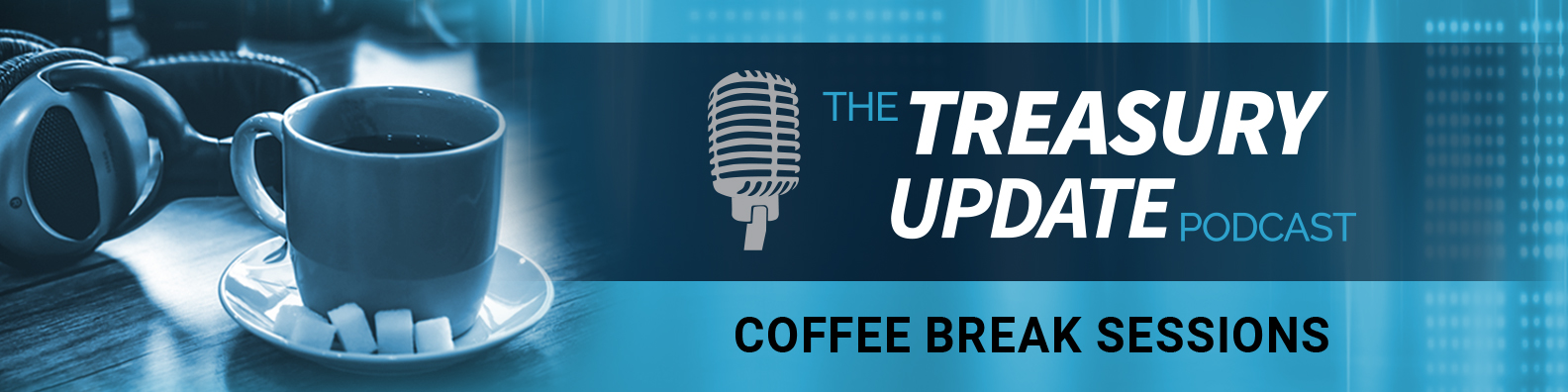
Session 93
Coffee Break Session: What Is Data Extraction?
Join us for a quick coffee break as we discuss data extraction in treasury operations. In this episode, we’ll explore how AI is reshaping the process, tackle common challenges, highlight AI-driven innovations, share success stories, and address trust and reliability concerns.
Host:
Jonathan Jeffery, Strategic Treasurer
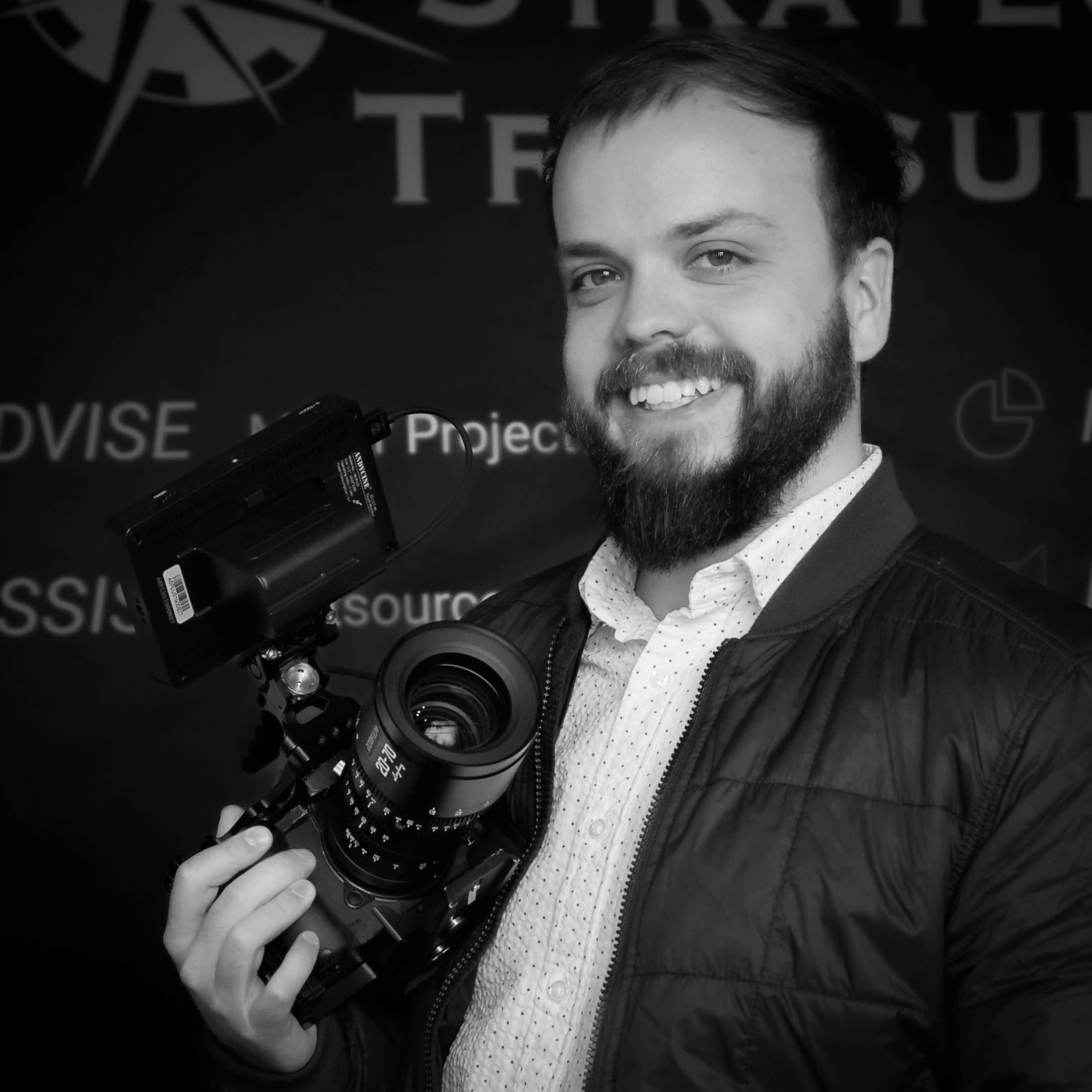
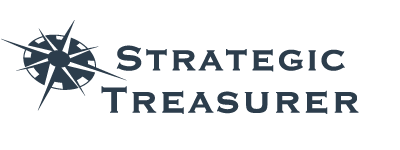
Speaker:
Craig Jeffery, Strategic Treasurer
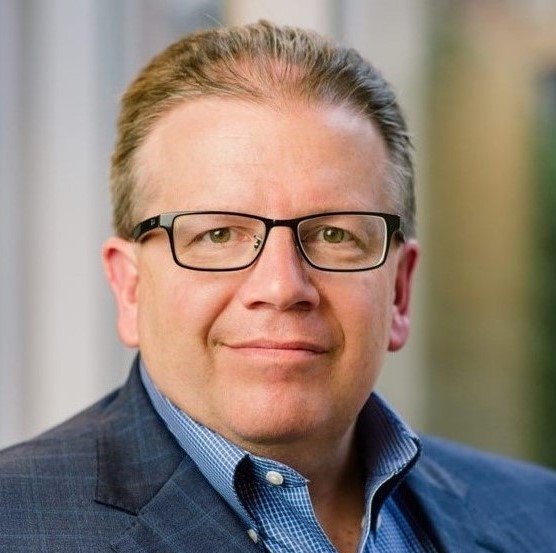
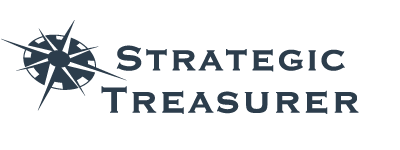
Episode Transcription - (Coffee Break Session Series) - Episode 93 - What Is Data Extraction?
Jonathan Jeffery 00:02
Welcome to the Treasury Update Podcast, Coffee Break Sessions presented by Strategic Treasurer, the show where we cover foundational topics and core treasury issues in about the same amount of time it takes you to drink your cup of coffee. I’ll be your host, Jonathan, media production specialist here at Strategic Treasurer. So sit back, relax and enjoy the show. I am here with Craig Jeffery, and I got a question for you, Craig.
Craig Jeffery 00:28
Yeah, go ahead.
Jonathan Jeffery 00:29
What do you call getting data out of systems, sources, or even people’s heads?
Craig Jeffery 00:35
What do I call it? I think, I think the title of this episode is data extraction. So I would call it data extraction.
Jonathan Jeffery 00:42
Okay, do you want to elaborate on what data extraction is?
Craig Jeffery 00:45
We need data to function. And so extraction is pulling data out, and then you put it into some other location, sometimes people refer to that is consuming the data, or even ingesting the data, that’s been a much more popular term of light. And it’s really the process of making data accessible. Extraction and access is really what we’re thinking about here.
Jonathan Jeffery 01:07
So the data is unusable before it’s extracted.
Craig Jeffery 01:11
If you can’t see it, you can’t really analyze it or, or put it through your model. So the idea is making it available to where you want to use it, you know, in a spreadsheet, in a business intelligence tool, into your, you know, into a data lake, wherever.
Jonathan Jeffery 01:27
Gotcha. So this is part of the technology and data series on the Coffee Break Sessions. So let’s talk about the technology behind it a little bit. What, what ways are AI helping with data extraction?
Craig Jeffery 01:39
We can define data in three different ways. One is structured data, think of rows and columns, or databases, semi structured, where there it might be, there’s some element to the structure, like a system log file that says what was done and what was changed to unstructured data. And you can think of news articles as an example of unstructured data where AI is helping, we’ve never really had much of a hard time with structured data. When you get to semi structured and unstructured data, being able to pull that, you know, maybe something semi structured, put it into some type of structure that’s easier to use, or unstructured data, like news articles to determine what the Feds going to do with their interest rates. This is certainly allows organizations to use this technology to roll through massive amounts of unstructured data and and gain some insights gained some gain some information that that’s going to be useful for planning their cash flow, looking at risk, or perhaps detecting different types of exposure changes.
Jonathan Jeffery 02:43
Okay, so it’s helping sift through some of the data that you don’t even need to look at.
Craig Jeffery 02:47
If you haven’t, you can’t like you couldn’t hire enough people to look at it. And so allows you to work through that. Yeah. Of course it does. You know, if you use something like chat GPT, there’s a sense of AI to do that, right. That was part of your question. You can go and ask for ask for things. And it misses elements. It doesn’t it doesn’t categorize something. I remember I did this to check, you know, what were the reserve requirements for the US. And I couldn’t remember when things changed. There wasn’t necessarily a table I could find. So I, I sent it off on its mission. And it came back and it didn’t have some information. It’s like, I knew there was a 12% reserve requirement, one point in time, etc. And I said, What about these rates? And then it looked and said, oh, sorry, and I’m not sure why it said sorry, but went through it, I guess it hunted a little more deeply found some other data points and said, Oh, yeah, for this period, there was this rate. If I didn’t have some knowledge of some of these changes, I might have been content with that initial search. Same thing. If you have bad data, or miss formatted data, and you do a query and you don’t pull up all of the companies, you’re expecting to hear all of the names, you might have more exposure to a particular counterparty, but something’s classified differently. And so therefore, you miss it. So there’s always the issue of you might miss some data, you might miss some information. But hopefully, as we look at these and manage these processes more thoroughly, there’s ways to help get more and more data. But that’s always the challenge that you might miss something, you might miss something that’s important. Yeah.
Jonathan Jeffery 02:53
Does it miss stuff? Eventually, it’ll get to a point where you ask it to do something for you. And we’ll come back with some clarifying questions I haven’t seen or it’s doing that yet. But that might that might get you into much more detail. Like you were looking for a specific number correct. And it couldn’t find the information. But if it had come back and said, give me more information on what you’re looking for in a category or whatever, then it knows where to look specifically.
Craig Jeffery 04:45
Well, if we’re talking about AI and extracting data or pulling out data and doing some research, you know, the the ability for the machine to run through data would be should be much more thorough. So if I’m looking to see correlate shins are causation between planned temperature changes, what will that do to our how much oil we buy? And what about the price of oil over time, those may be things I’m concerned about from a treasury perspective. But there might be some other elements that create preceding factors or cause and the fact that you could have tried to find other correlations that I don’t necessarily know what to think about. It’s like, I know that consumption is related to temperature outside because there’s heating and cooling that goes on, well, maybe there’s another factor about the economic variables and changes there, there might be three other factors that I don’t think about. But you know, the idea of I want to extract data, and I want to do some analysis, I might be able to find more correlations by having it go and hunt and see if it can find some mathematical or logical connection that I don’t necessarily think about all in half of a second. I think that’s gonna take a little more than half of a second, I know sometimes when we ask us is like, it’s churning through lots of data. So from an AI perspective, it’s churning through a lot of data. But you’re right, it’s like, it’s ridiculously fast, you know, compared to, you know, we have interns, and it used to be assigned interns, these tasks where they got to plow through a ton of data. Well, that’s not what we assigned them now so much, because you can have the system churn through stuff, but they do it way faster, they usually do a better job the first till the interns really, really well trained, and they understand the domain. So I think that’s a that’s another area that’s changed and challenge what we’re doing.
Jonathan Jeffery 06:33
Do you have any practical examples of ways that people are working this into their technology?
Craig Jeffery 06:38
So probably the most broad based practical example of data extraction, I would say would be where companies want to leverage the power of data lakes, they have cloud native technology, they want to leverage business intelligence tools on top of data, lakes and other sources, the ability to extract this type of data, put it in a location that can be used well, you know, allows for some of those areas, they talked about, you know, fraud detection, other anomalous value of doing anomaly detection, for quality control, example, or anything that’s out of the ordinary, also for pattern detection and trends, like forecasts that could be for accounts receivable, and treasury. Now on the accounts receivable side, and Treasury side, there’s quite a bit happening now, it’s primarily been done by the technology firms that have built the AI functionality. And the tools are to run things on an unattended basis at at a detail level at an individual client, let’s say for accounts receivable to look and make projections at a company, by company basis, a customer by customer basis, based upon their payment trends, which is different in the past, it used to be you’d have to do a statistical analysis against the law of large numbers and what existed there find some type of level of fit into what you would expect for the data. And now it’s, it’s able to grind through at a detailed level. So that’s helping with forecasting for AR for overall treasury, those things are being done largely being done by the tech vendors, and companies are starting to adopt that.
Jonathan Jeffery 08:21
Gotcha. So you said something about AI missing? Well, it’s sifting through this data, it can miss information. But is the information that it’s giving you always reliable? Or does it just make stuff up? Does it come to wrong conclusions?
Craig Jeffery 08:36
When you send something to look for data depends on the parameters that are used. And the tools like if you just run a query is going to pull something back or not, you might set some tolerances to, to retrieve the data. But yeah, I think anybody knows, you know, from playing around with Excel, you know, certain types of lookup type functionalities, you know, is the exact match or is it close? And knowing what can result, you know, it gives you something that’s close, as opposed to you’re only looking for something exact. And knowing those differences matters. However you query for that information. And, you know, depends on what the tool is trying to do. Will it give you something close will give you something general that’s true from the use of Excel two different tools, whether it’s, you know, using Python, or using some type of AI tool to gather data? Is it trying to extrapolate or triangulate something? But you just have to be careful on knowing what the range is and how those how they operate.
Jonathan Jeffery 09:34
So is it going to work when someone makes a decision, and then later on, it doesn’t go so well, and they go to their boss and say, the AI told me to do it?
Craig Jeffery 09:44
Well, you know, I don’t know that. AI systems has surpassed humans and the ability to find scapegoats to accuse or blame others, like nobody could have predicted this to happen. Well, it’s not true people predicted certain things are done. Data was really bad. And we didn’t, we didn’t expect it to be that bad. Well, data is always bad, we always expect it to be bad. And it’s, you know, there’s all kinds of assumptions on the quality of data we have. And so this is another tool. Hopefully, we use these types of tools to better triangulate and total thing. So the idea of how do you reconcile the data that you get? How do you know if it’s reasonable? You know, it’s reasonable, because you have deep experience in that area? You know, it’s reasonable, because data sources are very trustworthy. You know, the data from a file, perhaps from a file that came in perhaps, is trustworthy, because there’s hashing, mathematically, there’s no way it could have been changed, or there’s control totals based upon certain fields are added up. There should be ways to check and validate some some items to make sure it’s not just directionally correct. But from a magnitude perspective, it’s it’s pretty close, it’s in the neighborhood.
Jonathan Jeffery 10:56
All right. So data extraction is pulling data from multiple different sources, and treasuries using AI to make that process extremely quick and save man hours. And any final thoughts on this, Craig?
Craig Jeffery 11:12
The only thought is, you know, you know, as you’re asked some questions, it made me it made me think about the idea of, you know, trust, but verify, you know, triangulate, do reasonability checks, make sure we’re, we’re understanding data and the tools we use, you know, and it brings me back to a really old example, I remember, sometimes we would look at spreadsheets, people would run, and there would be some kind of total they’d put on there. And the total would be off by I can’t remember what the amount was off. I was like, was off by 40,000. And this was probably the most common mistake. And if you looked at the numbers, yeah, don’t click like, why is it off. And this was oftentimes in the far right column at the very top of the spreadsheet that put a date in a numerical value as it was a date. And so the bottom, they would run the, the sum, and it would capture the date, they would add it as a date, so it’d be off by a certain number. And so these are unintended consequences of not thinking through, you know, if I run it to the top of the page, I include other datasets. That type of situation is we always have to be careful. It’ll vary how that plays out in different types of technology and different in different days and ages. But we always have to be a little bit skeptical, check, make sure it’s reasonable, make sure it fits, and we’ll do better when that’s the case.
Jonathan Jeffery 12:29
Great. Well, thank you for joining us, Craig. And for our listeners, tune back in every first and third Thursday of the month for another episode of the Coffee Break Sessions.
Announcer 12:43
This podcast is provided for informational purposes only, and statements made by Strategic Treasurer LLC on this podcast are not intended as legal, business, consulting, or tax advice. For more information, visit and bookmark StrategicTreasurer.com.
- Treasury & Risk Management Systems
- Treasury Aggregators
- Supply Chain Finance & Cash Conversion Cycle
- Enterprise Liquidity Management
Learn more about these technologies and evaluate some of the top vendors in each industry.