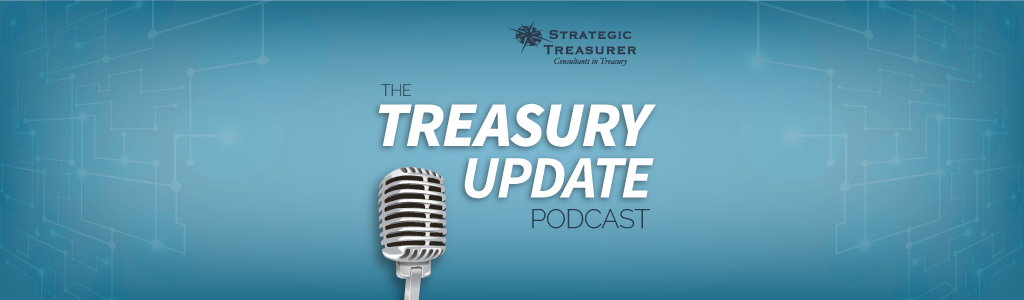
Episode 174
Cash Forecasting & Visibility Survey Results
Host:
Craig Jeffery, Strategic Treasurer
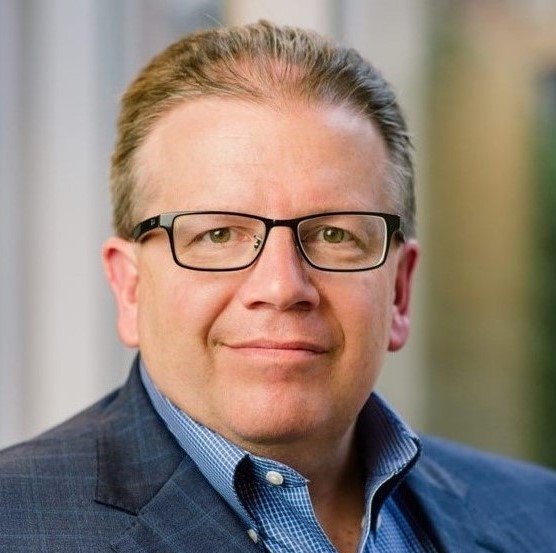
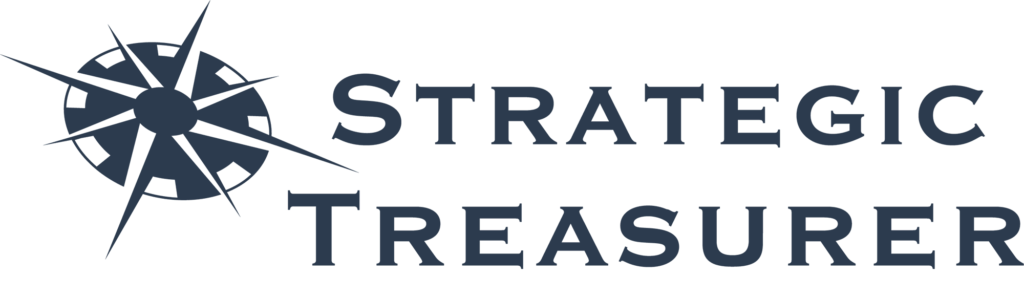
Speaker:
Roger Comins, GTreasury
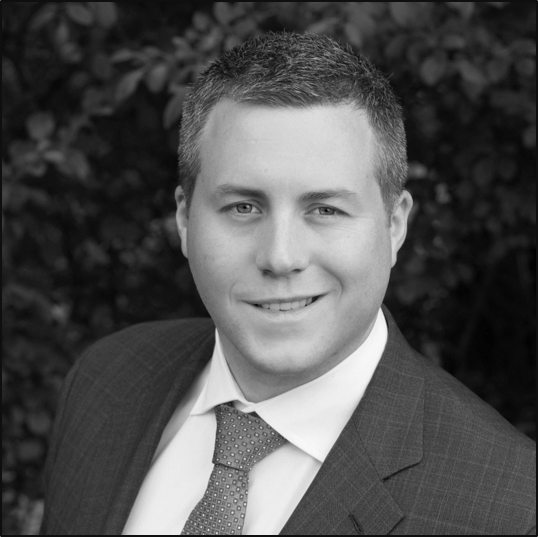
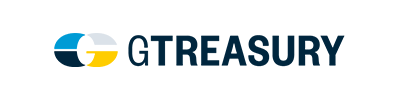
Subscribe to the Treasury Update Podcast on your favorite app!
Episode Transcription - Episode 174: Cash Forecasting and Visibility Survey Results
INTRO 0:05
Thanks for tuning in to the Treasury update podcast. If you’re attending AFP this year, we’d love to see you. Please stop by Strategic Treasurer’s AFP booth number 1927 and tell us you listen to our podcast for a Starbucks gift card as a thank you. We hope to see you there. Enjoy today’s program. Welcome to The Treasury Update Podcast presented by Strategic Treasurer, your source for interesting treasury, news, analysis, and insights in your car, at the gym, or wherever you decide to tune in. On this episode of the podcast, host Craig Jeffrey joins Roger Comins, Vice President of Product Management at GTreasury to discuss some key findings of the 2021 Cash Forecasting and Visibility survey results. Topics of discussion centered around the top pain points for the modern treasury department, innovative technologies that help, and disruptions that add to the challenges. Listen in to learn what others in the treasury and finance industry are experiencing and doing to handle today’s challenges.
Craig Jeffery 1:21
Welcome to The Treasury Update Podcast. This is Craig Jeffrey, today’s host, and I’m here with Roger Cummins, who’s VP of Product Management at GTreasury. Roger, welcome to The Treasury Update Podcast.
Roger Comins 1:33
Yeah, thanks, Craig. Thanks for having me. Very excited for the topic at hand here in forecasting.
Craig Jeffery 1:39
Well, thank you and GTreasury for underwriting this year’s edition of Cash Forecasts and Visibility survey. Before we get into to the meat, I want to point people to a couple assets. You can look in the show notes and see where you can download the survey results report and related information. So that’s one place to go. Just a couple items just to highlight a few of the key findings. There’s quite a few of them. We’ll be talking about some of them. Here’s a couple points that I found particularly interesting it’s this is good news for those with a TMS generating cash positions is three times harder if you aren’t using a TMS but that was pretty interesting. So, we broke out the groups for those who are using things like Excel or other tools versus a TMS, and we found that 33% of those who use other methods indicate it’s hard to generate a cash position. Second, and we’ll talk about this, Roger and I will discuss some of this is that we call the rise in the forecasting machine, so this is using AI and machine learning to help generate forecasts. And right now, the percentage of organizations that responded to the survey was down around 6%. They’re using it for forecasting now. The expectation based on results is that within two years that number will exceed a quarter of companies, so 27%. So, moving from 6% to 27% in a couple years very very interesting. So, this is usually tech that’s delivered through the TMS. And then the third one I wanted to point out just to whet your appetite is that half of firms or 48% have an extremely difficult time generating forecasts. And before those, everyone says we knew that we didn’t need a survey, I think it’s interesting to note that it’s half and why that’s interesting is it’s under a quarter of firms have an easy time generating forecast versus those that have a difficult time. So, it’s a two-fold factor. That’s some additional calibration there, twice as many companies have a difficult time doing this versus those that have an easy time. And since this is so important, as we’ll get into, that’s going to be interesting to see how this changes over time. So, Roger, thanks, thanks again for underwriting this year’s research and I wanted to get into the topic about the challenge of forecasting. So, we just looked at some numbers, 48% having a difficult time versus 23% easy time. Why is it so difficult? It’s always seems, at least the last four or five years, it’s a top concern it takes a lot of time, it’s not accurate, and it’s hard. Why is that?
Roger Comins 4:34
That’s an interesting question. I’d be curious to at some point, see if there’s any correlation to size of company for those who found it easy. But why it’s hard, I mean, in my mind is a couple things. So, one, to do it well, you need in somewhat of a unique manner, when you think about treasury processes, you need data from every area of the business. Right? As we know, budgeting itself is difficult and all the data that goes into budgeting, you’re typically going to need for forecasting. And to my question on maybe size of company correlating as we know as a company is going to grow in size. So is it going to grow and some of the systems to make sense of it all and so I think with forecasting, the fact that you need data from each area of the business and add to it that you’re going to have different systems where that’s maintained. Ideally, maybe it’s all in an ERP, but it’s at least in different modules of an ERP. It can be hard to automate that to get it into a central repository to dissect and analyze and make sense of it all. And so, what I think a lot of folks probably find is, one they might actually fail to get automation from all the various areas and that’s okay, that’s to be expected and you’re not alone. So, then we kind of tend to rely then on data entry by humans, which we know can lead to inaccuracies. Maybe simpler processes, where I’ll take numbers from last year and then a certain percentage to them just across the board in a sweeping manner, which again, it’s likely going to be a little less accurate than getting data directly from some of the sources. So, forecasts is probably one of the toughest nuts to crack when you think about all the processes that the treasury team has to manage and it’s to me it’s because of all those data sources they have to centralize.
Craig Jeffery 6:36
You know with that, as we ran into the pandemic, there was a much greater emphasis on running more forecast additional models with different assumptions from you know, rosy, realistic, negative, hyper-negative, and the frequency that those had to occur sped up in so many companies. We did a lot of research with the Treasury Coalition, which you were part of, and we saw how that created a lot of challenges to more frequent forecasts with more models. That certainly stresses the human side of activities. What are we learning from either the pandemic or just general increased expectations around forecasts?
Roger Comins 7:24
Yeah, no, I think we’re hearing a lot of the same even directly from our customer base, you know, where it might have been a weekly or monthly process and review with their senior management team before, the need for forecasts has become an almost daily process, which does put additional strain on the resources especially if that automation isn’t in place. I’ll also and I know we’ll talk about how it impacts different structured treasury groups in a minute, but the other pandemic has definitely, from a process perspective, added strain, and then, if you think about one of the points I made before just about if there are some of these data sources, you don’t have automating you’re kind of relying on past data to kind of make a sweeping assumption about the future well the pandemic has made it so that you know, maybe your past year isn’t very telling of what this current year is. So, I think people are struggling to fully understand some of the market factors that play into their numbers they’re projecting. And so, I would think that that’s probably a little bit more impactful on some of the medium to longer term horizons that they’re looking at, you know, short term it’s probably more just about frequency of publishing a forecast. But yeah, as you’re trying to plan for kind of the medium and long term, the pandemic’s is going to make it very difficult to rely on some of the past data that you might have. That’s what we’re hearing from our customers. It’s interesting to hear as you guys have done some of your surveying and outreach that you’re seeing a lot of that too.
Craig Jeffery 9:05
So, we have the, I don’t know if you want to call it the environmental challenge of the pandemic having a feedback loop on to forecasting difficulties. I think the concerns about interest rates and inflation are certainly much on people’s radar, certainly over the last couple of months, as it has moved up from very low levels to concerning rates of speed, maybe with those things in context and maybe leaving it out I wanted to get your thoughts on the different treasury organizations and the impact of being centralized or decentralized. Whether it’s decentralized different treasury centers that all report up to one area or different functions, what should we be thinking about with regard to how we organize for forecasting for the implications for forecasts?
Roger Comins 10:08
Yeah, no, absolutely. And maybe I’ll speak to it in terms of as a product person what I’ve, you know, we’ve learned through some of our customer research is really the difference of prioritized needs. When you think about a forecasting solution, centralized and in some cases, in most cases, it’s every type of organization, whether they’re centralized or decentralized, but centralized is going to prioritize that automation, that visibility of their data in one place, and that easy ability to do different kind of scenario analysis especially during the pandemic, right? You mentioned some of the scenarios, people are looking at, you know, some of the sunny day rainy day scenarios, but when you talk to some of the decentralized treasury organizations, and just to step back and think about what the difference between those two is with decentralized, you’ve got a, you know, a treasury center who’s relying on basically all of their entities to roll up their forecasted numbers to them and to manage all of that. So, it’s a little bit less about connecting to systems and a little bit more about needing a workflow to, you know, deal with multiple human elements and trying to centralize everything being rolled up from different entities across the company. So, things like review and approval structures being in place, making sure the data structures are all delivered in the same way so that you can have a composite view of everything. It’s kind of where the priority lies of a decentralized treasury. So, while they all need automation, as the pandemic stresses the frequency and urgency of accurate forecasting, I think it’s interesting being a product person talking to these two different types of treasury organizations where the decentralized really really needs that workflow to keep everyone on the same set of inputs and also to have that kind of review and approval structure in place. So, I know that’s very much in the frame of reference of systems, but hopefully that’s interesting.
Craig Jeffery 12:33
You know, this idea of a workflow where it might be personnel heavy. And to some extent, the workflow may feed into more automated tools, like machine learning, you know, is, you know, some of the problems of the real long-term problems that we see with forecasting as we’re getting data laid, its incomplete, people don’t know people that might be submitting it don’t care about it as much or more data is partial. And it creates this we have to spend a lot of time doing it. How much of the solution needs to fall into place with data cleanliness or using tools like machine learning to determine pattern detection? How do we solve these problems? Maybe I should just leave it at that.
Roger Comins 13:23
Yeah, no, it’s a great question. So, I think one thing I’ll just lead with is that machine learning definitely does not solve all the problems right. You mentioned, data cleanliness, machine Learning models are really only going to be as good as the data you feed them so that cleanliness is an important part. If anyone listening should kind of think of their own roadmap to success, whether you have ML or not machine learning or not you definitely need to first achieve that automation and cleanliness in my mind, but what I’ll say machine learning definitely helps with is where I made some points about if I’m having a hard time maybe pulling in a source of certain data and I might be just copying from a prior year, what ML does do an offer is a way to project that data by learning about your history and in a way that’s going to take into account certain factors that more of a simple averaging forward isn’t going to do, like understanding your spikes at starts of months end of month, certain months in general certain quarters right, all the seasonality aspects that your business might have. Machine learning is going to pick up on that and give you a more accurate projection than maybe some of the simple averaging that folks would do for those data sources that aren’t populated straight from the ERP. But again, it needs to learn from something and so that data cleanliness is an important part of your roadmap towards machine learning. If I can make some comments around just other aspects of what’s important for machine learning, and it may be I’ll even go so far as to say that systems still need to accomplish is really taking into account those like market data factors, market influencing factors, right? So during like a pandemic, especially we’ve already stated that your past data may not be telling of your current business. So, it’s so critical to also have that factoring of the different scenario analysis that you can do in a product instead of just relying on any kind of projection, even machine learning, so that you can see what some of the market influences might be on your cash flows. So, really bringing into account some of the you know, forward projections that would come off of like a risk modeling system you know, is probably the best you can get out of this right to bring it all together to actually look at like some of the cash flow at risk modeling, in conjunction with what you’re doing is really kind of the target state is so that you cannot just rely on a model that looks at the past but also takes current market influences into account. So, it’s a lot, there’s a lot for TMS’s to achieve to get this right. And I’d go so far as to say, you know, there’s still a lot to be done there for a lot of the TMS’s
Craig Jeffery 16:50
Okay, I’ve got a follow up for you on machine learning. There’s machine learning has a lot of skill in determining patterns. It seems like there’s two ways it can help with forecasting, one is seeing patterns to making projections and that certainly fits into the automate and clean the data so that you’re basing it off for the right, good data in. Does it also provide a lot of promise for detecting patterns where something you know on a quality control level, data you’re getting in for projections, maybe from other areas could be out of line with what’s expected, they’re anomalous, they fall outside the band and require attention. Is that a is that another area of opportunity? Or is that already being factored in?
Roger Comins 17:41
No, absolutely, I think that is an area of opportunity. It’s two different approaches to modeling, I would say, but they work very well in conjunction right. And what good would look like is it just like you said, right for a model to point out what data, if it’s going to factor out projections for each data set, which one may have some anomalies in it, some uncleanliness in the data like missing data throughout and even better yet, there’s a lot of approaches to data science that will take care of those things in the background, and you don’t even have to worry about as a user but yes, you know, a good model should make anything that needs the human interaction needed, you know, available and present to the user, as you know, like an alert. So absolutely agree with you.
Craig Jeffery 18:43
Yeah, I know there’s a lot a lot of ground to go on that but just seeing how the percentage of companies that are pursuing it, want to use it with their different providers, from ERP systems to treasury management systems to other tools, definitely seems to be a growing chorus of an opportunity for these types of tools. Before I ask you about any final thoughts, I wanted to gauge any quick reaction for you on these items. This will be a speed round of to say a couple things about forecasting. So cash forecasts will increase in importance for 59% of companies and this is the one year outlook, 27% say significantly more important 32% more important. This is this like five years of increased importance. When will cash forecasting stop being one of the top two items of you know what treasury wants to improve on?
Roger Comins 19:44
Hopefully it doesn’t, you know, I think with the current times, we’re in that’s not to be unexpected, right? You know, having an accurate forecast is more critical than ever, having solid forecasting and then risk management are probably going to be two very top concerns, you know, for the foreseeable future, for sure. I don’t know that I have an answer that when it will stop, I don’t think it will.
Craig Jeffery 20:10
Alright, the second one is Excel forecasters are more dissatisfied than their TMS and the ERP peers. So, those forecasting with their treasury management system or ERP are far less dissatisfied than Excel. So, 29% of Excel forecasters are dissatisfied compared to just 8% of the forecasters who use a TMS and ERP, that is a 21 point gap. The simplicity of Excel means it’s a very, very common tool, the most common tool, but the satisfaction gap or the dissatisfaction gap, in this case, 21-point gap is significant. Does this signify an early stage of more people doing forecasting in Excel, I’m sorry, in their TMS or in their ERP.
Roger Comins 21:06
So, what I would think I’m going to make a leap on a correlation there, like you said, Excel is very flexible, so I don’t think they’re probably dissatisfied with that aspect. I would say it’s likely that unless you’ve built a very lockdown model, anyone can mess with your numbers at any time because, you know, there’s not a workflow and data integrity with Excel. So, I would guess it’s one on that but two, that, just the correlation is that if you’re still in Excel, you’re likely lacking on some of those automations of the different data sources. So, I’m going to make that leap that is one of the main reasons there that they’re dissatisfied is because they have not yet achieved some of the automation that you would have as part of your TMS project. That’s going to really cut down on that manual effort letting you move into more of a strategic role.
Craig Jeffery 22:04
Fair enough. And the next the next one, this will be the last one. I know this is unfair to springs these on you. The two top areas for plans to spend heavily on tech are treasury systems and cash forecasts and 41% plan significant spending over the next year, 40% plants spend significantly on cash forecasting over the next year and so like if we just jumped back three years both of these numbers represent an increase. So, treasury systems 38% in 2018 to 41% this year, so that’s a significant investment. And then we went from 33% in cash forecasts in 2018 to 40% this next year. Now trying to think of a clever question to stump you on that. Is that good news for treasury management system providers?
Roger Comins 23:00
Yeah, I mean, I think it is and I think people are, especially as again like I keep hitting on the point of the pandemic has changed process for them. Forecasting is rarely probably for anyone listening now, a monthly task, it’s probably increased in frequency. So, the importance for a system is more than ever, in that automation so I think it is good news for TMS’s and you know, I think anyone listening is probably either already has explored or is exploring a TMS if they haven’t already and would find that you know, the systems out there are going to add that value to their daily process that’s going to help them
Craig Jeffery 23:48
As we wrap up this, this session on forecasting and visibility and in this year’s survey results. Any final thoughts for the audience?
Roger Comins 23:59
Yeah, I’ll go back to kind of the road mapping for those listening to do to do on your part. Whether you’re looking for a system or just to improve even an Excel process. Seeking out you know the automation, the various data sources you need to produce an accurate forecast is so critical to transforming your process again system are not, and to getting satisfaction out of out of your daily process in general. So, spend time with the areas of the organization where you need data from and to understand the systems you need data out of and don’t try to do it alone, right? Engage the right technical resources to help because again, system or not, you’re going to need partnership from your folks within your organization, technical folks, folks from the other side of the office of the CFO, so you know, start those conversations early.
OUTRO 25:06
You’ve reached the end of another episode of The Treasury Update podcast. Be sure to follow Strategic Treasurer on LinkedIn, just search for Strategic Treasurer. This podcast is provided for informational purposes only, and statements made by Strategic Treasurer LLC on this podcast, are not intended as legal, business, consulting, or tax advice. For more information, visit and bookmark strategictreasurer.com.
Related Resources
2021 Cash Forecasting & Visibility Survey Reports
This survey, underwritten by GTreasury, sought to understand the current trends in forecasting methods and technology, visibility and reporting, and technology use. What are your peers’ practices surrounding these activities today? What kinds of technology do they use now, and what are they planning to invest in soon? This report seeks to explain what others in the treasury and finance industry are experiencing and doing to handle this challenging set of activities.
Why TMS Should Be the Cornerstone of Your Treasury Transformation
Host Craig Jeffery joins Ashley Pater, Chief Product Officer at GTreasury, to cover questions from their webinar on why a treasury management system (TMS) should be the cornerstone of your treasury transformation. They share valuable insights and examples on how to transition treasury operations to a modern and efficient technology stack, complete with automation and integration that allow organizations to focus on strategic tasks.